Table of Contents
Introduction
Global Life Science Analytics Market size is expected to be worth around USD 20.9 Billion by 2033 from USD 9.7 Billion in 2023, growing at a CAGR of 8.0% during the forecast period from 2024 to 2033. In 2023, North America led the market, achieving over 56.1% share with a revenue of USD 5.4 Billion.
The global life science analytics market is primarily driven by the extensive investment in drug therapy research and development by pharmaceutical and biotech companies, alongside contract research organizations. These analytical tools are critical for identifying treatment patterns early, crafting personalized therapies, enhancing revenue analysis, and optimizing the patient care pathway.
The rapid growth of the market is further propelled by the increasing digitalization within hospitals and research centers, which enhances the efficiency of data handling and supports the operational needs of these institutions. The affordability of data management systems plays a crucial role in facilitating this development.
High volumes of patient data are managed daily in countries like the United States, India, and China, where advanced data-driven technologies provide significant advantages over traditional methods by enabling faster analysis of diverse healthcare data sets including patient records, treatment effectiveness, inventory, and staff management.
The COVID-19 pandemic had a positive impact on this market, leading to an uptick in research and development activities as companies rushed to develop new vaccines and treatments. For instance, in 2020, the healthcare segment of Cognizant reported a revenue increase to USD 4,852 million, up from USD 4,695 million in 2019. This growth was attributed to heightened demand for pharmaceutical services and strong software license sales.
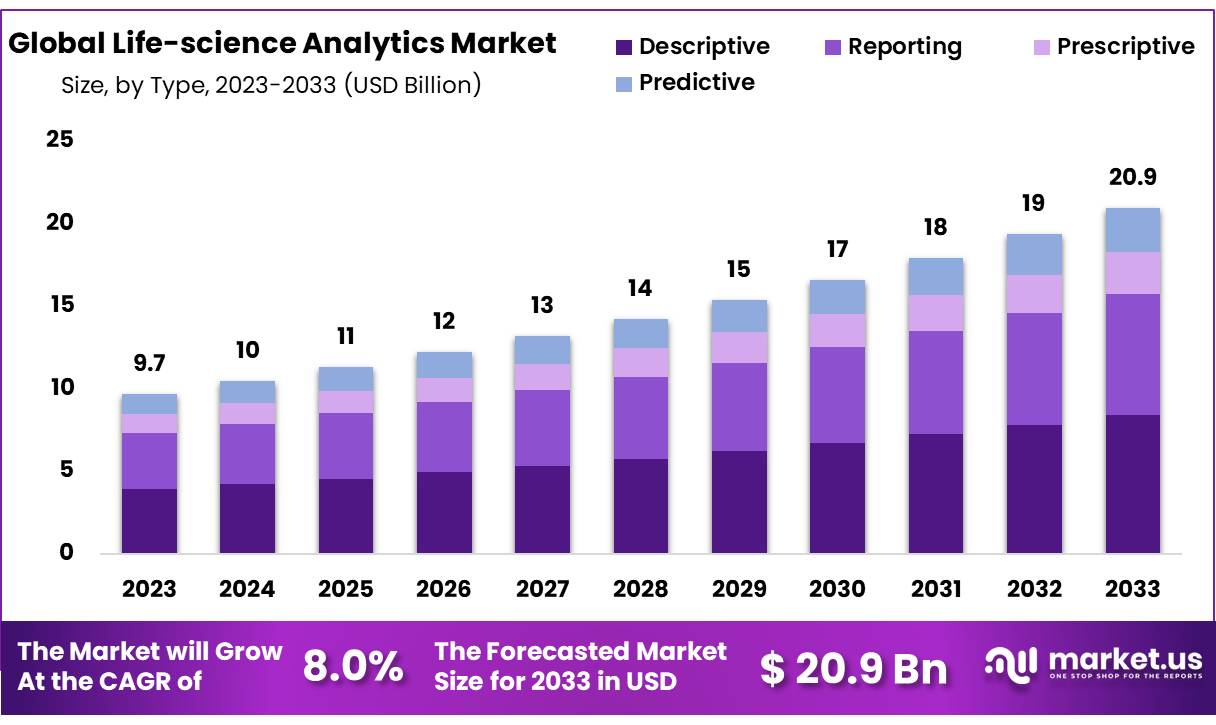
Key Takeaways
- Market Size: Life Science Analytics Market size is expected to be worth around USD 20.9 Billion by 2033 from USD 9.7 Billion in 2023.
- Market Growth: The market growing at a CAGR of 8.0% during the forecast period from 2024 to 2033.
- Type Analysis: In 2023, the descriptive type segment will hold the largest market share at 62.4%.
- Component Analysis: In 2023, the services segment accounted for the highest revenue share of 62.4%.
- Application Analysis: The sales and marketing segment accounted for the largest share, over 39.3%.
- End-Use Analysis: In 2023, the pharmaceutical segment dominated the market by accounting for the highest market share of 51.8%.
- Regional Analysis: North America dominated the market by accounting for the highest revenue share of 56.1% in 2023.
Life Science Analytics Statistics
- Employment and Salary Trends
- High Placement Rate: Within six months of graduation, 84% of analytics graduates secure full-time positions, highlighting the strong market demand for their skills.
- Competitive Salaries: In the U.S., analytics roles command an average base salary of $109,000, with salaries ranging from $60,000 to $187,000 based on experience levels.
- Data Scientist Roles: Data scientists represent about 44.4% of job placements in analytics, marking it as a predominant role within the field.
- Student Demographics and Background
- U.S. and International Representation: Of the analytics students, 63% are U.S. citizens and 37% are international, coming from 21 different countries.
- Educational Backgrounds: 40% of these students have a technical background in fields such as computer science, data science, statistics, machine learning, or artificial intelligence, preparing them for data-centric roles.
- Teaching Fellowships: 15% of Harvard’s data science students take on teaching fellowships, contributing to the spread of advanced data skills.
- Industry Placement
- Financial and Tech Sectors: 46% of analytics professionals find roles in the financial services and technology sectors, underlining the significant reliance on data analytics in these areas.
- Healthcare, Consulting, and Engineering: Another 30% are employed in consulting, healthcare, and engineering, indicating the broad applicability of analytics across various disciplines.
- Geographic and Salary Disparities
- International Average Salaries: The average salary for international placements is approximately $76,667, showing notable geographic salary differences.
- Placement in Northeast U.S.: 21% of advanced analytics roles are based in the Northeastern U.S., where salaries tend to be competitive.
- Program Growth and Work Experience
- Increased Enrollment: Since 2020, there has been a 30% increase in enrollment in U.S. university analytics programs, reflecting growth in this sector.
- Median Work Experience: Students typically enter these programs with 1.5 years of work experience, suggesting that many are at an entry-level stage in their careers.
- Role Specifics
- Data Engineering and Manipulation: Around 40% of roles involve data engineering and manipulation, underscoring the essential technical skills required in analytics.
- Data Science as a Dominant Role: Data science positions make up about 45% of analytics placements, affirming its high demand across industries.
- Advanced Degree Holders
- Postgraduate Representation: Approximately 13% of students in analytics programs hold advanced degrees, enhancing their expertise and role preparedness.
Life Science Analytics Application Analysis
- Sales & Marketing Support: Life science analytics enhances sales and marketing efforts by optimizing omnichannel marketing strategies and utilizing AI-driven insights. This technology enhances customer segmentation and engagement, leading to more personalized and effective marketing tactics. Predictive analytics are also used to forecast market trends, which supports the creation of targeted marketing campaigns and boosts sales productivity.
- Research & Development (R&D): In R&D, analytics are crucial for accelerating drug discovery and managing clinical trials, thereby enhancing the speed and efficiency of research outcomes. The use of predictive analytics is vital for identifying potential future discoveries and innovations, making this sector the fastest-growing area in analytics application within life sciences due to its significant impact on organizational pipelines.
- Supply Chain Analytics: In life sciences, supply chain analytics improve operational efficiency by providing insights into demand forecasting, inventory management, and logistic optimizations. These analytics facilitate a more streamlined supply chain management system, reducing costs and ensuring the timely delivery of medical products and services.
- Regulatory Compliance: Analytics help life science companies maintain compliance with regulatory standards throughout various product development and marketing stages. This is essential for keeping operations within legal and ethical boundaries, thus preserving market credibility and preventing legal issues.
- Pharmacovigilance: The use of analytics in pharmacovigilance improves drug safety monitoring by analyzing extensive data on adverse drug reactions. This enhances the early detection of potential risks, increasing patient safety and meeting global regulatory standards.
- Pre-Clinical Trials: Analytics are also employed in pre-clinical trials to simulate and model outcomes. This predictive capability aids in assessing the efficacy and safety of drugs before advancing to more costly phases of clinical trials, reducing both time and financial investment in drug development.
Emerging Trends
- Generative AI Growth: Generative AI is gaining traction in life sciences for its potential in data analysis and predictive modeling. Although still in the experimental phase, it promises significant analytical advancements by enabling organizations to predict outcomes and enhance operational efficiency more accurately.
- Shift to Industrialized Data Science: Companies are transitioning from personalized, “artisanal” methods of data modeling to an industrialized approach. This shift involves the use of Machine Learning Operations (MLOps) systems to streamline the creation, deployment, and management of data models, facilitating a more efficient data science workflow.
- Data Products Dominance: Life science organizations are increasingly creating “data products” that package analytics in a reusable format. Managed by data product managers, these tools are designed to improve decision-making by integrating data with analytics and AI for both internal and external applications.
- Role Diversification in Data Science: The field of data science is diversifying, introducing new roles such as data engineers and machine learning specialists. This expansion reduces the reliance on highly specialized data scientists and makes analytics more accessible to non-technical users.
- AI in Population Health: Advanced analytics are being leveraged to enhance population health management by identifying health trends within communities and enabling predictive care, which improves health outcomes on a large scale.
- Increased Data-Driven Clinical Decisions: In healthcare, life science analytics play a crucial role in clinical decision-making by identifying patterns in patient data, optimizing treatment plans, and improving patient outcomes.
- Enhanced Data Privacy and Security through Blockchain: Blockchain technology is being increasingly utilized to secure sensitive healthcare data, enabling the safe sharing of patient information while maintaining compliance with privacy standards.
- Predictive Modeling for Operational Efficiency: Predictive analytics are employed to enhance healthcare operations, such as improving appointment scheduling, reducing no-show rates, and optimizing staff deployment, potentially saving millions annually.
- Integration with Wearables: The integration of data from wearable devices, such as heart monitors and fitness trackers, is becoming a critical source for real-time health analytics, aiding in early disease detection and ongoing patient monitoring.
- AI in Error Reduction: AI tools are increasingly used in medical fields to reduce errors and enhance diagnostic accuracy, particularly in radiology, where AI can detect complex conditions early.
- Enhanced Real-World Evidence (RWE) Usage: Life sciences companies are expanding their use of real-world evidence, integrating data from diverse sources like electronic health records (EHRs) and social determinants to improve patient care and facilitate the development of new therapies.
- Data Visualization for Improved Decision-Making: The adoption of data visualization tools in healthcare is enabling practitioners to easily interpret large data sets, assisting in the identification of trends and the efficient allocation of resources.
- Value-Based Care Transition: Analytics are pivotal in the shift from fee-for-service to value-based care models, which focus on improving patient outcomes through preventive care, predictive insights, and outcome-based reimbursement strategies.
Use Cases
- Predictive Risk Assessment: Analytics tools forecast potential patient risks, such as the development of diabetes, enabling healthcare providers to offer early interventions and tailored prevention strategies that mitigate long-term health complications.
- Real-Time Health Monitoring: Wearable devices gather health data in real time, facilitating continuous monitoring of conditions such as heart disease. This enables immediate alerts to healthcare providers for necessary interventions.
- Patient Engagement: The use of data from wearables increases patient involvement in managing their health. For instance, linking incentives to health goals boosts engagement and encourages patients to adopt healthier lifestyles.
- Opioid Abuse Prevention: Analytics help identify patients at risk of opioid abuse by analyzing key indicators, leading to preemptive measures and more effective interventions to curb opioid misuse.
- Enhanced Imaging Analysis: Machine learning algorithms are employed to scrutinize medical images, like MRIs, to uncover subtle patterns that enhance the speed and accuracy of diagnoses, particularly for conditions like cancer.
- Telemedicine Expansion: The combination of analytics and video conferencing technologies facilitates remote patient monitoring, expanding healthcare access to underserved communities and helping reduce hospital admissions.
- Emergency Room Optimization: Sharing data among emergency rooms minimizes redundant tests and unnecessary visits by efficiently utilizing patient records across different locations, optimizing resource use.
- Staffing and Scheduling: Predictive analytics forecast patient inflow and optimize staffing levels, thereby improving hospital operational efficiency and ensuring adequate care during peak periods.
- Preventing Medication Errors: Analytics minimize medication errors by monitoring patient histories and potential drug interactions, aiding healthcare providers in making safer prescription decisions.
- Clinical Trial Optimization: Advanced analytics enhance the efficiency of clinical trials by swiftly identifying patient responses and potential complications, reducing the time it takes to bring new treatments to market.
- Epidemic Tracking: Analytics assist public health officials in monitoring and responding to disease outbreaks, such as influenza or COVID-19, by supporting timely and effective public health interventions.
- Cost Management: Analytics are crucial in optimizing spending within healthcare facilities, helping to maintain a balance between providing quality care and achieving financial sustainability by closely monitoring operational costs and resource allocation.
- Predictive Insights for Chronic Care: By analyzing data from lifestyle choices, genetic information, and health records, analytics can identify patients who may benefit from chronic care management programs, enhancing quality of life and reducing hospital readmissions.
Conclusion
The life science analytics market is experiencing robust growth driven by substantial investments in R&D and an increased emphasis on digitalization across healthcare facilities. This trend is complemented by a surge in data-driven decision-making, which significantly enhances patient care efficiency and treatment personalization.
The market’s expansion is also fueled by the evolving landscape of technological innovations such as AI and machine learning, which streamline operations and improve outcomes across the healthcare spectrum. As such, life science analytics are proving indispensable for fostering advancements in drug development, patient care, and healthcare management.
Discuss Your Needs With Our Analyst
Please share your requirements with more details so our analyst can check if they can solve your problem(s)
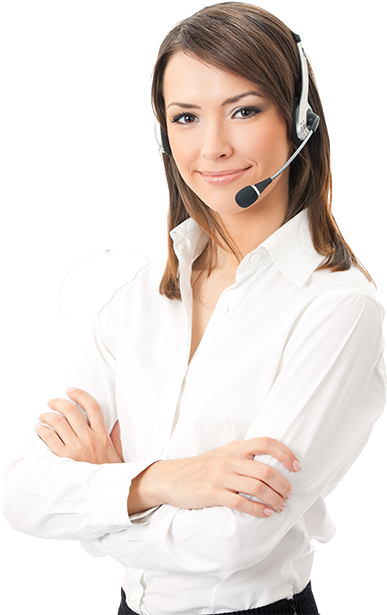