Table of Contents
Overview
New York, NY – April 28, 2025 – The Global AI In Predictive Toxicology Market size is expected to be worth around USD 4,964.3 Million by 2033 from USD 360.1 Million in 2023, growing at a CAGR of 30.0% during the forecast period from 2024 to 2033.
Artificial Intelligence (AI) is transforming predictive toxicology, offering a faster, more accurate, and cost-effective approach to assessing chemical safety and potential toxicity risks. Predictive toxicology traditionally relied on animal testing and labor-intensive laboratory methods. However, AI-driven models now enable the analysis of vast chemical datasets, allowing for early identification of toxicological effects before clinical trials or human exposure.
Using advanced machine learning algorithms, AI can predict toxic responses based on chemical structures, biological interactions, and genomic data. This technology improves decision-making in drug development, environmental safety, and chemical manufacturing by significantly reducing the need for animal testing and expediting regulatory approvals. AI models also help in understanding mechanisms of toxicity at the molecular level, enhancing the precision of risk assessments.
According to the U.S. Food and Drug Administration (FDA) and the National Institute of Environmental Health Sciences (NIEHS), integrating AI tools into toxicology workflows supports safer product development and aligns with global initiatives to minimize animal use in research. As industries increasingly adopt AI for predictive toxicology, the field is poised for substantial growth, driving innovations in pharmaceutical, environmental, and consumer safety sectors worldwide.
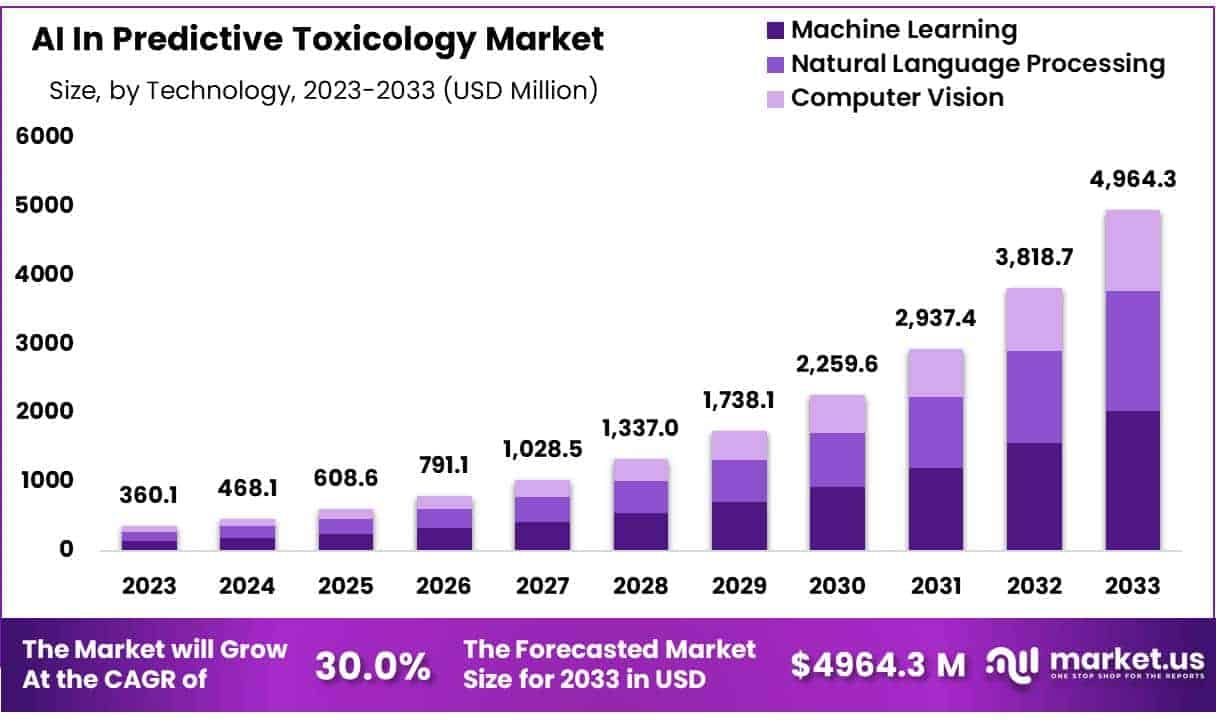
Key Takeaways
- Market Size: The AI in Predictive Toxicology market is projected to reach approximately USD 4,964.3 million by 2033, increasing from USD 360.1 million in 2023.
- Market Growth: The market is anticipated to grow at a robust compound annual growth rate (CAGR) of 30.0% during the forecast period from 2024 to 2033.
- Technology Analysis: Machine learning technologies led the market in 2023, capturing around 41% of the total market share.
- Toxicity Endpoint Analysis: Genotoxicity emerged as the leading toxicity endpoint in 2023, accounting for approximately 35% of the market share.
- Component Analysis: Solutions dominated the component segment in 2023, contributing to 61% of the overall market share.
- End-User Analysis: Companies represented the largest end-user group in 2023, holding about 53% of the market share.
- Regional Analysis: North America led the global AI in Predictive Toxicology market in 2023, securing nearly 44% of the total revenue share.
Segmentation Analysis
Technology Analysis: The AI in Predictive Toxicology Market is witnessing significant growth, driven by advanced technologies such as machine learning, natural language processing (NLP), and computer vision. In 2023, machine learning led the market with a 41% share, supporting complex biological data analysis and toxicity prediction. NLP enhances decision-making by rapidly analyzing scientific literature and toxicology databases. Meanwhile, computer vision automates histopathological image analysis, improving precision and supporting regulatory compliance while reducing reliance on traditional animal testing methods.
Toxicity Endpoint Analysis: In 2023, genotoxicity dominated the AI in Predictive Toxicology market, capturing 35% of the share. This highlights its importance in evaluating substances for potential genetic mutations or cancer risk. AI tools increasingly predict genetic damage early in drug development, enhancing safety. Hepatotoxicity, neurotoxicity, and cardiotoxicity are also critical endpoints. AI applications in these areas improve early detection of organ damage, minimize animal testing, and streamline the pharmaceutical industry’s regulatory approval processes.
Component Analysis: Solutions represented the dominant segment of the AI in Predictive Toxicology market in 2023, accounting for 61% of the total share. The surge in demand for AI-driven platforms that accurately predict chemical toxicity has accelerated solution adoption. These platforms integrate machine learning algorithms with vast biological databases, enhancing toxicity prediction capabilities. Services, including consulting and technical support, also play a vital role. As companies seek to optimize drug discovery, demand for AI solutions and services is expected to rise steadily.
End User Analysis: In 2023, companies accounted for 53% of the AI in Predictive Toxicology market, reflecting their widespread adoption of AI tools in early-stage drug development. AI models support high-throughput screening, improving drug discovery efficiency and safety outcomes. The chemical and cosmetics industries are also leveraging AI to ensure product safety and regulatory compliance. Additionally, research organizations and sectors like agriculture and food safety are adopting AI applications, highlighting the technology’s versatility and accelerating market expansion.
Market Segments
By Technology
- Machine Learning
- Natural Language Processing
- Computer Vision
By Toxicity Endpoints
- Genotoxicity
- Hepatotoxicity
- Neurotoxicity
- Cardiotoxicity
By Component
- Solution
- Services
By End User
- Pharma and Biotechnology Companies
- Chemical and Cosmetics
- Research Organization
- Others
Regional Analysis
In 2023, North America accounted for approximately 44% of the global revenue share in the AI in Predictive Toxicology market. This dominant position can be attributed to the region’s robust pharmaceutical industry and the increasing need for efficient drug development processes. Pharmaceutical companies across North America are progressively adopting AI technologies to enhance predictive toxicology capabilities, aiming to improve safety assessments and streamline research operations.
The integration of AI in predictive toxicology enables companies to accelerate drug discovery timelines and optimize research and development activities. These technologies contribute to significant cost reductions and operational efficiencies, reinforcing their importance in the competitive pharmaceutical landscape. Moreover, the region’s strong focus on innovation and technological advancement continues to drive the adoption of AI-based solutions within toxicological studies.
This trend highlights the industry’s ongoing commitment to embracing cutting-edge technologies to maintain a competitive advantage. The evolution of AI is expected to further revolutionize predictive toxicology, creating new avenues for growth and technological innovation across North America. As the pharmaceutical sector increasingly prioritizes safer and faster drug development, the role of AI in predictive toxicology will become even more critical in the coming years.
Emerging Trends
- In Silico Modeling and Simulation: AI-driven in silico models are increasingly utilized to simulate biological processes and predict toxicological outcomes. These models enable researchers to assess the potential toxicity of compounds without physical experimentation, thereby saving time and resources. The U.S. Food and Drug Administration (FDA) has been exploring such models to enhance regulatory science and safety assessments of FDA-regulated products.
- Integration of Multi-Omic Data: The amalgamation of genomics, proteomics, and metabolomics data through AI facilitates a comprehensive understanding of toxicological mechanisms. This integrative approach aids in identifying biomarkers and understanding the pathways involved in toxicity, thereby enhancing the predictive accuracy of toxicological assessments .
- Explainable AI (XAI): The adoption of Explainable AI techniques is gaining traction to address the “black box” nature of traditional AI models. XAI provides transparency in decision-making processes, enabling researchers and regulators to understand the rationale behind toxicity predictions, thus fostering trust and facilitating regulatory acceptance.
Use Cases
- Drug Safety Assessment: AI models are employed to predict adverse drug reactions by analyzing chemical structures and biological data. For instance, the FDA’s AI4TOX program aims to develop AI tools to support regulatory science and strengthen the safety review of FDA-regulated products.
- Chemical Risk Evaluation: The Environmental Protection Agency (EPA) utilizes machine learning algorithms to assess the toxicity of environmental chemicals. These models analyze vast datasets to predict potential health risks, thereby informing regulatory decisions and public health policies.
- Developmental Toxicity Screening: AI has been applied to automate the assessment of developmental toxicity. For example, AI models have been used to analyze micro-CT images of rabbit fetus skeletons to detect developmental anomalies, demonstrating the potential of AI in automating complex toxicological evaluations.
- Exposure Assessment: AI technologies are increasingly applied to analyze complex toxicological data and predict chemical or drug hazards. This application aids in understanding disease mechanisms, identifying new therapeutic targets, and informing risk assessment processes.
Conclusion
AI is fundamentally reshaping predictive toxicology by offering faster, more accurate, and cost-effective methods for assessing chemical safety. The technology’s integration into pharmaceutical, chemical, and regulatory processes is enhancing early detection of toxicological risks, minimizing reliance on animal testing, and expediting drug development.
With machine learning leading technological adoption and North America dominating regional growth, AI is poised to drive significant advancements across industries. Emerging trends such as in silico modeling, multi-omic data integration, and explainable AI are further strengthening predictive capabilities. As adoption rises, AI will continue to transform toxicology, delivering safer products and accelerating innovation globally.
Discuss your needs with our analyst
Please share your requirements with more details so our analyst can check if they can solve your problem(s)
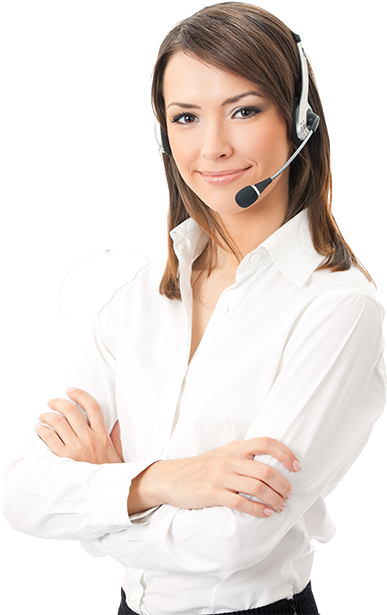