Table of Contents
Introduction
Global AI In Medicine Market size is expected to be worth around USD 156.8 Billion by 2033 from USD 13.7 Billion in 2023, growing at a CAGR of 27.6% during the forecast period from 2024 to 2033. With a market share over 41.7%, North America held a strong lead in 2023, reaching US$ 5.71 Million in revenue.
The growth of AI in healthcare is driven by advancements in AI technology, increasing adoption of AI-based diagnostic and treatment solutions, and a growing focus on personalized medicine. The rising prevalence of chronic diseases and the demand for efficient healthcare delivery systems further contribute to market expansion, creating significant opportunities for stakeholders.
Advancements in AI technology are revolutionizing medical diagnostics and treatment. AI algorithms now analyze complex medical data with greater accuracy and efficiency, particularly in radiology, pathology, and genomics. These systems can identify patterns and anomalies often overlooked in manual analysis, significantly enhancing diagnostic capabilities.
Personalized medicine is also gaining prominence, supported by AI’s ability to process large volumes of patient data. AI-driven insights enable tailored treatments, offering more effective and targeted therapies. This approach is critical in managing chronic diseases and improving patient outcomes.
Additionally, the increasing prevalence of chronic conditions such as diabetes and cardiovascular diseases drives the need for AI-powered healthcare solutions. These technologies facilitate early detection, ongoing monitoring, and efficient management of chronic diseases, thereby reducing the strain on healthcare systems and enhancing patient care.
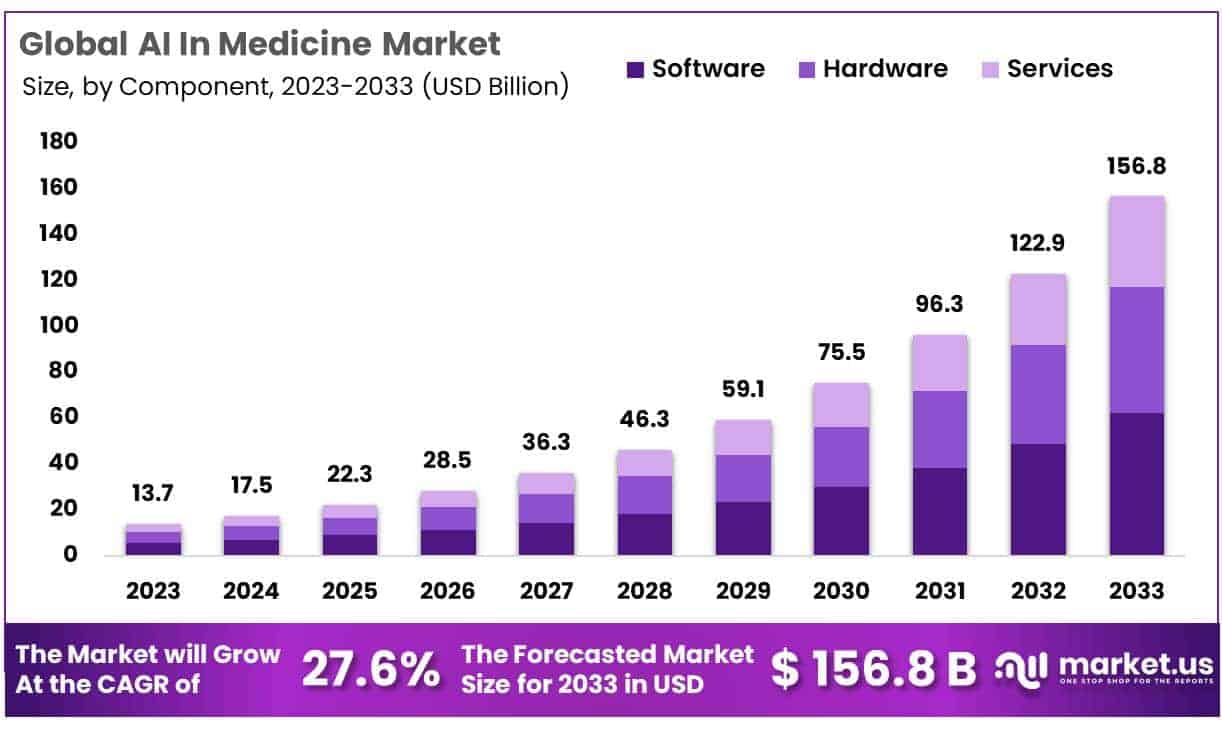
Key Takeaways
- Market Size: The AI in Medicine market is expected to grow significantly, with a projected worth of USD 156.8 billion by 2033, marking a substantial increase from USD 13.7 billion in 2023.
- Market Growth: The market growth is anticipated to occur at a robust Compound Annual Growth Rate (CAGR) of 27.6% over the forecast period from 2024 to 2033.
- Component: The market is segmented into hardware, software, and services. In 2023, the software segment held the largest market share, contributing 39.7% of the total revenue.
- Technology: Machine learning emerged as the top revenue generator, capturing 43.6% of the market share in 2023.
- Application: The largest market share in 2023 was held by the Patient Data & Risk Analysis segment, accounting for 39.5% of the market revenue.
- Regional Analysis: North America: Led the market in 2023, commanding a market share of 41.7%, driven by high AI adoption rates, government investments, and the rising incidence of chronic diseases.
- Asia Pacific: Expected to experience the highest CAGR during the forecast period, fueled by growing IT and healthcare infrastructure, increasing investments, and favorable government policies.
- Drivers: Technological advancements, particularly in machine learning, natural language processing, and computer vision, are enhancing analysis, interpretation, and processing capabilities, leading to applications such as medical imaging interpretation, predictive analytics, and personalized treatment recommendations.
- Restraints: Data security and privacy concerns surrounding sensitive healthcare data pose challenges, as maintaining confidentiality and integrity is paramount.
- Opportunities: Personalized healthcare and precision medicine offer avenues for tailor-made treatment regimens and workflow optimization, utilizing AI technologies to improve therapeutic outcomes and operational efficiencies.
AI in Medicine Technology Analysis
- Machine Learning (ML): ML algorithms analyze vast datasets to identify patterns and make predictions, significantly contributing to medical diagnostics and treatment planning. For instance, ML models have been developed to predict patient outcomes and assist in early disease detection. The U.S. Food and Drug Administration (FDA) acknowledges the transformative potential of AI/ML in medical devices, emphasizing their role in analyzing large amounts of data and supporting robotic-assisted surgical devices.
- Natural Language Processing (NLP): NLP enables computers to understand and interpret human language, facilitating the extraction of meaningful information from unstructured clinical notes and medical literature. The National Institutes of Health (NIH) has developed AI tools like TrialGPT, which uses large language models to efficiently identify and rank relevant clinical trials for patients based on their medical and demographic data.
- Context-Aware Computing: Context-aware computing involves systems that can sense and respond to their environment, enhancing patient monitoring and personalized care. By integrating data from various sources, such systems can provide real-time support tailored to individual patient needs, improving outcomes and efficiency in healthcare delivery.
- Computer Vision: Computer vision allows machines to interpret and process visual information, playing a crucial role in medical imaging. AI systems have demonstrated superior accuracy in detecting conditions like skin cancer from images compared to human dermatologists. A study published in the Annals of Oncology reported that an AI system using a deep learning convolutional neural network detected skin cancer more accurately than dermatologists.
AI in Medicine Key Statistics
- Improved Diagnostic Accuracy: AI-powered diagnostic tools enhance detection rates, achieving an 85% improvement in identifying certain cancers compared to traditional methods.
- Reduction in Diagnostic Errors: AI in medical imaging reduces diagnostic errors by up to 30%, potentially saving billions annually in healthcare costs.
- Personalized Medicine Effectiveness: AI applications in personalized medicine increase treatment effectiveness for chronic diseases by 20%.
- Telemedicine Growth: AI-driven telemedicine platforms experienced a 40% usage surge during the COVID-19 pandemic, improving remote patient monitoring capabilities.
- AI Chatbots in Healthcare: By 2025, AI chatbots are expected to handle 75% of healthcare queries, reducing the workload for healthcare providers.
- Adaptive Algorithms in Clinical Settings: Adaptive AI systems in clinical use improve diagnostic accuracy by 15% through continuous learning from new data inputs.
- Cost Savings in Healthcare: AI technologies are projected to save up to USD 150 billion annually in the United States by 2026 through enhanced efficiency and error reduction.
- Regulatory Approvals: Regulatory frameworks are advancing, with the FDA approving over 50 AI-based medical devices within the past three years.
- Efficiency in Drug Development: AI in drug development reduces discovery time by 25% and costs by 30%, streamlining the development process.
- Predictive Analytics in Disease Models: AI-based predictive analytics improve disease outbreak modeling, increasing accuracy by 20%.
- Integration into Clinical Workflows: Collaborative efforts show that 70% of new AI tools require seamless integration into existing clinical workflows for effective adoption.
- Hospital Readmission Reduction: AI-driven remote monitoring solutions reduce hospital readmission rates for chronic disease patients by 50%.
- Addressing AI Biases: Efforts to reduce bias in AI systems have resulted in a 35% increase in the use of inclusive data sets, promoting equitable healthcare delivery.
Emerging Trends in AI for Healthcare
- Theranostics Integration The combination of diagnostics and therapy, termed *theranostics*, is transforming cancer treatment. AI enhances this approach by utilizing patient-specific data for precise diagnosis, tailored treatments, and effective follow-up care, particularly for cancers like prostate and neuroendocrine tumors.
- Multi-Cancer Detection Tests Innovations like multi-cancer detection (MCD) tests are being developed to screen multiple cancer types through a single blood test. These technologies enable earlier detection, improving treatment outcomes and survival rates.
- AI-Driven Clinical Trials The National Institutes of Health (NIH) is advancing clinical trials that systematically assess emerging cancer screening technologies. These trials ensure the effectiveness of innovations across diverse populations and support evidence-based screening guidelines.
- AI in Diagnostic Imaging AI algorithms are revolutionizing imaging technologies like MRI and CT scans, enhancing tumor detection and characterization accuracy. This is particularly useful in defining the extent of cancers, such as prostate cancer, beyond traditional imaging capabilities.
- Regulatory Advancements The FDA is establishing clear guidelines for developing AI technologies in healthcare, focusing on ensuring the safety and efficacy of AI-driven diagnostic tools and therapeutic applications.
- Virtual Health Platforms AI-powered virtual health platforms are increasingly utilized in telemedicine and remote patient monitoring. These platforms provide real-time health data analysis and facilitate virtual consultations, improving healthcare accessibility and efficiency.
- AI in Mental Health AI applications in mental health include chatbots and digital tools that enhance service delivery. These innovations address gaps in provider availability and improve patient engagement, adherence, and access to mental health support.
- Ethical and Bias Considerations Ensuring equitable healthcare through AI involves addressing biases in datasets. Efforts focus on improving inclusivity and quality in AI training data, reducing potential disparities in diagnostics and treatments.
AI Use Cases in Healthcare
- Enhanced Diagnostic Imaging AI-driven imaging improves tumor detection and characterization. For instance, AI refines prostate cancer diagnoses in MRI scans, offering earlier and more precise identification of the disease.
- Cancer Theranostics AI supports theranostics by combining diagnostic and therapeutic processes to target cancers like prostate and neuroendocrine tumors. This ensures precision in treatment and improved monitoring of therapy effectiveness.
- Multi-Cancer Detection Tests AI-powered MCD tests screen for various cancers using a single blood sample. Early-stage cancer detection through these tests enhances treatment effectiveness and increases survival rates.
- Personalized Medicine By analyzing genetic profiles and patient histories, AI tailors treatment plans to individual needs. This personalized approach improves therapy outcomes and efficacy in chronic disease management.
- Telemedicine and Remote Monitoring AI-powered telemedicine platforms provide real-time health insights and enable virtual consultations, making healthcare more accessible and efficient for patients worldwide.
- Predictive Analytics for Disease Outbreaks AI models predict disease outbreaks by analyzing data trends, enabling quicker responses and informed public health interventions to mitigate the spread of infections.
- AI in Mental Health Services Digital tools and chatbots driven by AI bridge gaps in mental health care, improving patient engagement and adherence while addressing challenges like provider shortages.
- Drug Discovery Acceleration AI accelerates drug discovery by analyzing molecular interactions and identifying potential candidates, reducing the time and cost required for new drug development.
- Regulatory and Safety Monitoring The FDA is creating frameworks to ensure AI-driven medical devices are safe and effective, focusing on software updates and lifecycle integration for enhanced patient safety.
- Surgical Precision AI assists surgeons by providing real-time data and predictive insights during procedures. This enhances decision-making and improves surgical outcomes, benefiting both patients and healthcare providers.
Recent Developments in AI in Medicine
- GE Healthcare: GE Healthcare unveiled the MyBreastAI Suite during the annual radiology and medical imaging conference in Chicago. This advanced AI-powered suite enhances breast cancer detection by using deep learning algorithms to analyze mammograms with improved accuracy and efficiency. By simplifying diagnostics and enabling early detection, this innovation highlights GE Healthcare’s dedication to leveraging AI for better patient outcomes and optimized radiological workflows.
- Atomwise Inc.: In March 2024, Atomwise Inc. launched AtomNet 2.0, an AI-driven drug discovery platform. Utilizing deep learning algorithms, this platform predicts the binding affinity of small molecules to target proteins, significantly accelerating the drug discovery process. By improving accuracy and efficiency, AtomNet 2.0 reduces both time and costs associated with developing new therapeutic candidates.
- Novo Nordisk A/S: In May 2024, Novo Nordisk acquired an AI-focused digital health company specializing in diabetes management solutions. The integration of these advanced AI technologies aims to enhance Novo Nordisk’s diabetes care portfolio by optimizing insulin dosing and glucose monitoring. This acquisition enables personalized treatment plans and real-time support for improved patient outcomes in diabetes management.
- Nano-X Imaging Ltd: In March 2024, Nano-X Imaging Ltd introduced the NanoX.ARC, an AI-enabled digital X-ray system. This innovative technology delivers high-resolution imaging with significantly reduced radiation exposure compared to conventional X-ray systems. The NanoX.ARC employs AI algorithms to enhance image quality, enabling more precise diagnostics and representing a major advancement in medical imaging.
- Medasense Biometrics Limited: In April 2024, Medasense Biometrics Limited launched the PainMonitor Pro, an AI-based pain assessment device. By analyzing physiological signals with advanced algorithms, this device provides objective, real-time pain measurements. PainMonitor Pro supports healthcare providers in making informed pain management decisions, thereby improving overall patient care and outcomes.
Conclusion
The integration of AI in healthcare is revolutionizing diagnostics, personalized medicine, and chronic disease management. Significant growth, projected at a CAGR of 27.6%, is driven by advancements in AI technology, increased adoption, and the demand for efficient healthcare solutions. Machine learning, NLP, and computer vision enhance diagnostics, while AI-powered tools improve efficiency in telemedicine, mental health, and drug development.
Regional opportunities, particularly in Asia-Pacific, and innovations in theranostics and multi-cancer detection underscore its transformative potential. Despite challenges like data security and biases, AI’s applications promise enhanced healthcare outcomes, cost savings, and operational efficiencies, marking a paradigm shift in modern medicine.
Discuss your needs with our analyst
Please share your requirements with more details so our analyst can check if they can solve your problem(s)
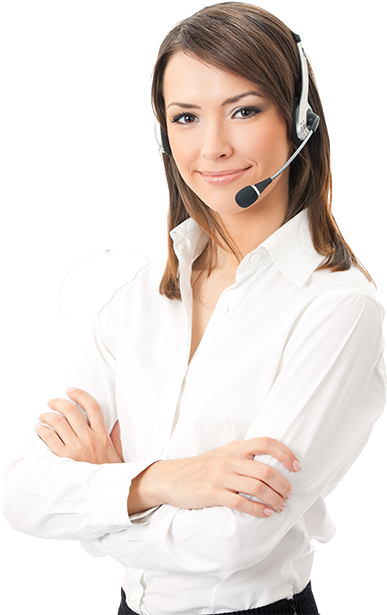