Table of Contents
Introduction
Global Artificial Intelligence in Drug Discovery Market size is expected to be worth around US$ 13.6 Billion by 2033 from US$ 1.2 Billion in 2023, growing at a CAGR of 27.5% during the forecast period from 2024 to 2033. In 2023, North America led the market, achieving over 60.1% share with a revenue of US$ 0.72 Billion.
This significant growth is driven by the expanding use of AI within the pharmaceutical industry, aimed at enhancing efficiency, reducing the time-to-market for new drugs, and addressing the complexities involved in drug development.
AI technologies are transforming the drug discovery field by providing solutions that quicken the process while increasing precision and cost efficiency. North America, especially the United States, is spearheading this market expansion, propelled by widespread adoption of AI in pharmaceutical research, robust healthcare infrastructure, and a strong emphasis on innovation. This is exemplified by notable partnerships between leading tech companies and pharmaceutical firms, which are crucial for leveraging AI to streamline drug discovery, optimize clinical trials, and advance the development of new therapeutics.
However, the market encounters challenges, particularly the significant costs linked to AI integration and the extensive infrastructure required, which may be daunting for smaller companies and underdeveloped regions. Despite these obstacles, the industry continues to progress, with software and machine learning technologies taking a dominant stance in the market due to their profound impact on data analysis and decision-making in drug discovery. This is especially pertinent in developing treatments for neurodegenerative diseases and in enhancing drug repurposing efforts, where AI’s analytical prowess can lead to innovative breakthroughs in therapeutics.
Further propelled by the COVID-19 pandemic, recent developments have highlighted AI’s indispensable role in drug discovery. The pandemic accelerated a shift towards digitalization in biomedical research, promoting rapid adoption of AI tools for data analysis, disease pattern recognition, and vaccine development. This trend reflects a larger movement towards digital transformation in healthcare, which is expected to persist in driving innovation and growth in the AI drug discovery market.
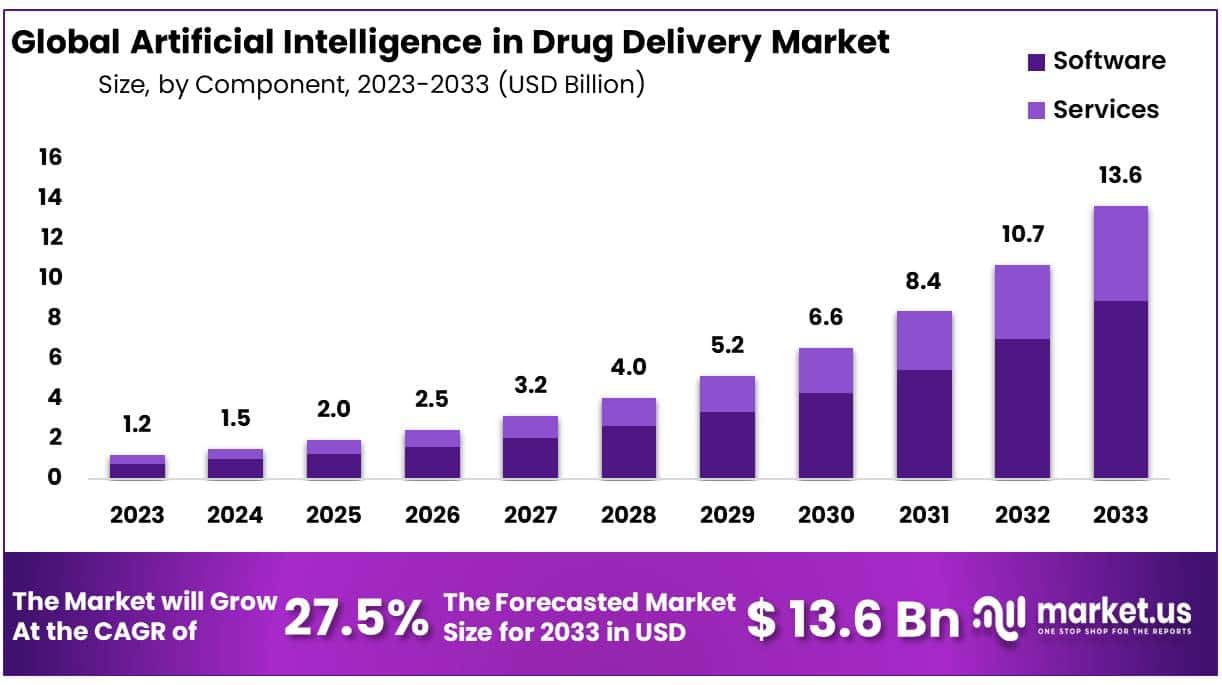
Key Takeways
- Global Artificial Intelligence in Drug Discovery Market was valued at USD 1.2 Billion. Between 2024 and 2033.
- This market is estimated to register the highest CAGR of 27.5%. It is expected to reach USD 13.6 Billion by 2033.
- The software leads the market by covering 65.4% share of AI in the drug discovery market.
- The machine learning dominates 52.7% market share of the technology segment due to its benefits.
- Neurodegenerative diseases was account 43.8% market share in 2023.
- Pharmaceutical and biotechnological companies cover 68.4% market share of AI in the drug discovery market.
- North America dominated the global artificial intelligence in the drug discovery market by accounting for a major revenue share of 60.1%.
- The pharmaceutical industry has witnessed significant growth in the use of artificial intelligence for drug discovery, enabling tasks like lead compound recognition, target validation, and drug structure optimization.
Key Statistics of AI in Drug Discovery Market
- Primary Market Users: Pharmaceutical and biotechnological companies are the primary users, holding a 68.4% market share in AI-driven drug discovery.
- Regional Leadership: North America dominates the global market, contributing 60.1% to the revenue.
- Market Expansion (2018-2024): The AI market in pharmaceuticals grew from $700 million in 2018 to an estimated $5 billion by 2024, reflecting significant growth.
- Investment Trends (2013-2018): Over $7.20 billion was invested in AI within the pharmaceutical sector through more than 300 deals between 2013 and 2018.
- Enhancing Drug Discovery: AI is leveraged to accelerate the traditionally long and costly drug discovery process, which typically exceeds a decade and incurs around $2.8 billion in costs.
- Clinical Trial Improvements: AI enhances clinical trials, which historically see only a 10% success rate, through better patient selection and monitoring.
- Sector Growth (2017-2024): The demand for AI in Drug Discovery Market is projected to grow by 40% from 2017 to 2024, indicating its transformative impact.
- Global Healthcare Expenditure: Annually, 6-7% of global GDP, amounting to $8.5 to $9 trillion, is spent on healthcare.
- Development Costs and Time: Launching a new drug typically costs over $1 billion and can take up to 14 years.
- Development Success Rate: Drug development, especially for cancer drugs, has a notably low success rate, with 97% of drugs failing during clinical trials.
- AI Investment Momentum: In the first half of 2021, AI startups in drug development garnered approximately $2.1 billion in funding.
- U.S. Leadership in AI: The U.S. hosts over half of the world’s AI companies focused on drug discovery.
- AI in Clinical Trials: AI is increasingly utilized in various drug discovery stages, including target identification, de novo molecular design, drug repurposing, and clinical trial efficiency.
- Data and Computational Advances: The last decade has witnessed a significant increase in medical data and advancements in computational hardware and deep learning techniques.
- AI Tools and Databases: AI tools like AlphaFold2 and DeepChem, along with databases such as PubChem, ChEMBL, and DrugBank, are integral in supporting the drug discovery process.
- FDA Approvals and AI: AI plays a growing role in expediting FDA drug approval processes by automating clinical trial data analysis.
- Predictive Model Development: AI is crucial for developing predictive models that enhance the speed and accuracy of drug-target interaction identification.
- Clinical Trials Milestones:
- February 2022: The first molecule discovered using AI began Phase I clinical trials.
- March 2022: Over 150 small-molecule drugs were in development by AI-driven biotechnology companies.
- March 2022: More than 15 drugs identified through AI methods advanced to clinical trial stages.
- January 2023: The first creation of de novo antibodies using solely computational methods was achieved.
- February 2023: The first Orphan Drug Designation for a drug discovered by AI was granted, showcasing AI’s potential in addressing rare diseases effectively.
Emerging Trends in AI-Driven Drug Discovery
- Machine Learning Algorithms for Drug Design: Increasingly, machine learning algorithms are employed in drug design, utilizing large datasets to analyze chemical compounds, biological targets, and drug properties to pinpoint potential drug candidates. Advanced deep learning techniques are particularly valuable, enhancing prediction accuracy and shortening drug discovery timelines.
- Predictive Analytics for Drug Safety Assessment: AI plays a vital role in forecasting and evaluating the safety of prospective drugs. By assessing data from clinical trials, adverse event databases, and genetic information, AI algorithms can identify potential safety issues early, allowing for the development of safer drugs and averting costly late-stage failures.
- High-Throughput Screening Automation: AI-driven automation is refining traditional high-throughput screening processes by quickly analyzing extensive compound libraries. Intelligent algorithms evaluate screening outcomes to prioritize promising candidates, thereby expediting drug discovery and reducing costs.
- Virtual Screening and Drug Repurposing: AI-powered virtual screening is employed to discover new uses for existing drugs. This method analyzes large databases of molecular structures and drug-target interactions to find existing drugs that can be repurposed, conserving time and resources by leveraging established data and clinical insights.
- Precision Medicine and Personalized Treatment: AI facilitates the development of personalized medicine by analyzing patient-specific data such as genomics and clinical records. Machine learning algorithms discern patterns and correlations in extensive datasets to tailor optimal treatment strategies for individual patients, potentially enhancing treatment outcomes and reducing healthcare costs.
Use Cases of AI in Drug Discovery
- Drug Target Identification and Validation: AI algorithms sift through vast biological and genetic data to identify and validate potential drug targets, pinpointing molecules or proteins crucial in disease progression for targeted drug development.
- Virtual Screening and Compound Design: AI-driven virtual screening methods are used to sift through large compound databases, employing machine learning and molecular modeling to predict drug molecules’ binding affinity and activity against specific targets.
- Drug Repurposing and Combination Therapy: AI analyzes extensive biomedical literature, clinical data, and molecular information to repurpose existing drugs for new therapeutic uses and suggest potential drug combinations to enhance efficacy or overcome resistance.
- Predictive Toxicology and Safety Assessment: AI is critical in predicting the safety and toxicity of drug candidates, analyzing data from chemical structures, biological assays, and adverse event reports to prioritize safer compounds for development.
- Clinical Trial Optimization: AI optimizes clinical trial designs and operations by analyzing diverse patient data, enabling targeted trials that reduce costs and accelerate drug market introduction.
Challenges in AI-Driven Drug Discovery
- Regulatory Hurdles: The rapid advancement of AI in drug discovery outpaces current regulatory frameworks, necessitating clearer guidelines to safely integrate AI into drug development processes.
- Data Quality and Accessibility: High-quality, diverse, and accessible data is essential for AI effectiveness. Data heterogeneity, privacy issues, and access limitations can hinder AI performance, requiring solutions to these data-related challenges.
- Ethical and Trust Issues: Building trust in AI models necessitates greater transparency in AI decision-making processes, demanding robust ethical guidelines and clear operational practices to enhance stakeholder confidence.
- Technical Limitations: Technical challenges such as scalability and interpretability of AI models continue to affect the predictive accuracy and reliability of AI in drug discovery, underscoring the need for ongoing technical enhancements.
Opportunities with AI in Drug Discovery
- Enhanced Drug Repurposing with AI: AI significantly streamlines the process of discovering new applications for existing drugs, offering a cost-effective alternative to new drug development and potentially providing quicker access to treatments.
- Streamlining Clinical Trials: AI transforms clinical trial management by assisting in participant recruitment, adaptive protocol design, and efficient monitoring, optimizing trial designs and outcomes.
- Predictive Analytics for Improved Drug Safety and Efficacy: AI’s predictive capabilities are crucial for enhancing drug safety and efficacy, supporting the development of safer, more effective pharmaceuticals by anticipating adverse effects and efficacy.
- Cross-sector Collaboration in AI and Drug Discovery: Collaborations between tech giants and pharmaceutical companies are intensifying, leveraging advanced AI technologies to address complex drug discovery challenges and drive innovation in the sector.
Key Players in AI-Driven Drug Discovery
- NVIDIA Corporation: NVIDIA is enhancing drug discovery with its BioNeMo platform, providing pre-trained biomolecular AI models to assist tasks such as protein structure prediction and small molecule generation. This platform, accessible via cloud APIs, is central to NVIDIA’s strategy, helping companies integrate AI into their drug discovery processes to speed up the development of new therapeutics.
- Microsoft Corporation: Microsoft uses AI to streamline drug discovery, notably in collaboration with Novartis. This partnership exemplifies Microsoft’s role in advancing AI applications in pharmaceuticals, using its AI innovations and computational power to reduce the time required for drug development by improving molecular structure predictions and optimizations.
- Cloud Pharmaceuticals: Specializing in AI-driven drug design, Cloud Pharmaceuticals uses proprietary AI technology to significantly cut the timeline from target validation to lead molecule development. A notable collaboration with GlaxoSmithKline focuses on designing small-molecule agents, showcasing the application of its AI solutions in large-scale pharmaceutical endeavors.
- Atomwise, Inc.: Atomwise employs its AtomNet platform, utilizing convolutional neural networks to accelerate drug discovery. By predicting bioactivity and optimizing lead compounds, Atomwise collaborates with industry giants like Sanofi to target complex biological markers, increasingly shifting towards developing its proprietary drug candidates.
- Schrödinger: Schrödinger’s use of computational platforms integrates physics-based simulations with machine learning to streamline the drug design process. Recent collaborations with companies such as Bristol Myers Squibb and Otsuka in neuroscience research demonstrate Schrödinger’s significant impact on expediting and refining drug development.
- BioSymetrics: BioSymetrics is advancing AI in drug discovery by integrating vast clinical and experimental data, which aids in target identification and the development of precision medicines. Its Contingent-AI framework is pivotal for rapid predictions of mechanisms of action, enhancing efforts in drug discovery and repurposing, particularly in fields like epilepsy treatment.
- Cyclica Inc.: Cyclica enhances drug discovery with its technologies such as MatchMaker and POEM, facilitating broad proteome analysis to identify potential drug interactions. This approach supports drug design, repurposing, and target deconvolution, with notable collaborations including those with Merck, emphasizing Cyclica’s role in advancing AI-driven drug discovery.
- IBM Watson: IBM Watson contributes significantly to AI-driven drug discovery with its advanced data analysis tools and natural language processing capabilities. These technologies help identify new drug targets and repurpose existing drugs, streamlining the discovery process and reducing development time and costs.
- BenevolentAI: Leveraging its Benevolent Platform™, BenevolentAI focuses on discovering novel drug candidates and validating new drug targets across various diseases. Its collaboration with AstraZeneca and efforts in diseases like idiopathic pulmonary fibrosis and systemic lupus erythematosus underscore its commitment to using AI to solve complex biomedical challenges.
Conclusion
The integration of AI in drug discovery is revolutionizing the pharmaceutical industry by accelerating the development of new therapeutics, enhancing precision, and reducing costs. Despite challenges such as high implementation costs and regulatory hurdles, AI technologies, particularly machine learning, are driving significant advancements in areas like drug repurposing, clinical trial optimization, and safety assessments.
North America remains the dominant region, with notable collaborations between tech companies and pharmaceutical firms fueling market growth. As AI continues to evolve, it promises to reshape drug development, streamline processes, and offer new opportunities for treating complex diseases, positioning the sector for substantial growth.
Discuss your needs with our analyst
Please share your requirements with more details so our analyst can check if they can solve your problem(s)
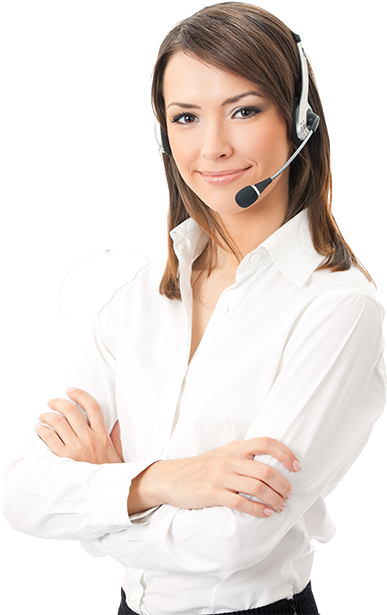