Table of Contents
Introduction
Global AI In Bioinformatics Market size is expected to be worth around US$ 136.3 Million by 2033, from US$ 3.8 Million in 2023, growing at a CAGR of 42.9% during the forecast period from 2024 to 2033. In 2023, North America led the market, achieving over 46.5% share with a revenue of US$ 1.7 Million.
This growth is driven by increasing demand for bioinformatics, decreasing sequencing costs, and significant funding from both public and private sectors for bioinformatics research. Technological advancements and strategic collaborations among leading players, such as Thermo Fisher Scientific, Illumina Inc., and Qiagen, are further fueling market expansion. These collaborations often focus on developing or upgrading bioinformatics tools to efficiently manage biological data essential for gene therapy, drug discovery, and personalized medicine.
Recent developments highlight the market’s dynamic growth, with investments accelerating genomic and proteomic data analysis. These advancements are critical for understanding disease mechanisms and identifying therapeutic strategies. AI-powered bioinformatics is revolutionizing the field by enhancing data analysis speed, facilitating discoveries of new disease pathways, and identifying potential therapeutic targets.
Despite its promising growth, the market faces challenges such as the lack of standardized data formats, the need for user-friendly tools, and the complexities of managing large biological datasets. However, with ongoing technological innovations and increased investments, these challenges are expected to be addressed, creating a robust environment for further market expansion.
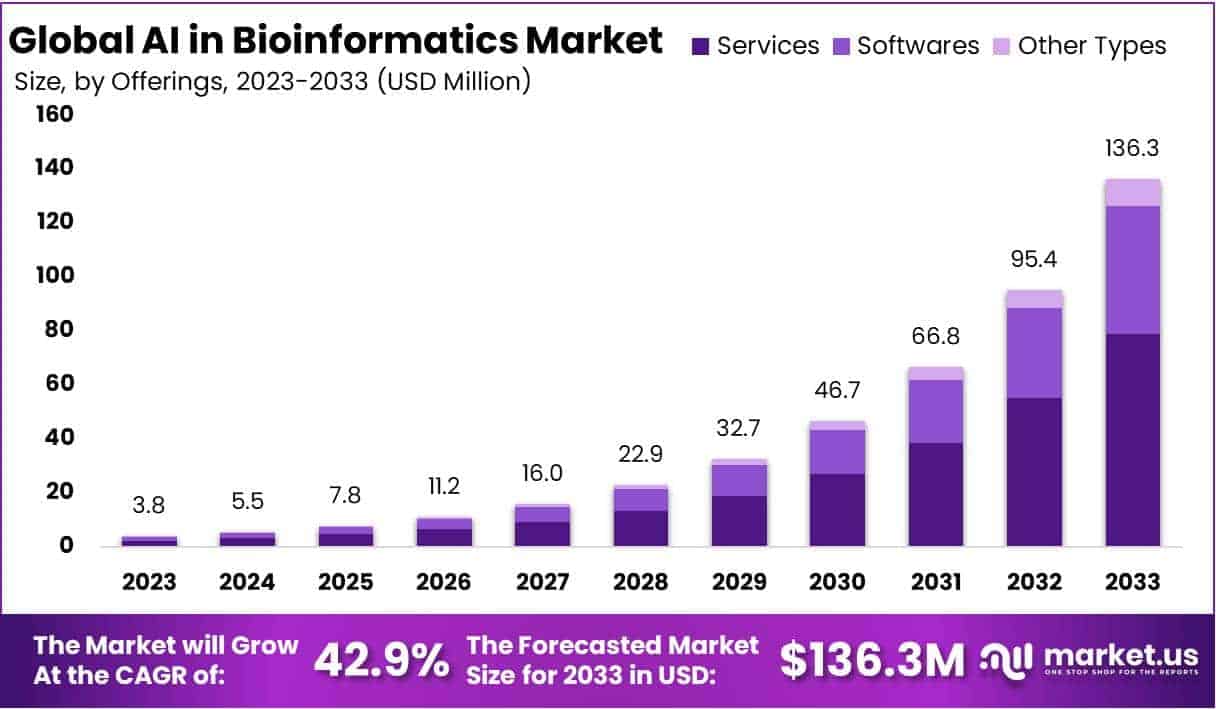
Key Takeaways
- The AI in Bioinformatics market is projected to expand significantly, reaching an estimated value of US$ 136.3 million by 2033.
- Starting from a base of US$ 3.8 million in 2023, this market is anticipated to experience a robust CAGR of 42.9%, forecast period from 2024 to 2033.
- In 2023, the analysis services segment significantly dominated, achieving a 57.8% share of the market, indicating its pivotal role in the industry’s operations.
- Machine learning emerged as the leading technology within the AI in bioinformatics sector, commanding a substantial 49.3% market share in 2023.
- The bioinformatics service segment showcased remarkable profitability, securing a dominant 61.5% market share in 2023, reflecting its critical importance.
- The genomic applications segment outperformed others in 2023, with its scaling advantages ensuring a leading 27.7% share of the market.
- The medical biotechnology sector excelled in 2023, capturing a notable 38.4% revenue share, highlighting its dominance in the market.
- Dominating the global AI in bioinformatics landscape, North America accounted for over 46.5% of the market, translating to a value of USD 1.7 million in 2023.
AI In Bioinformatics Statistics
- AI processes genetic data up to 100 times faster than traditional methods, enabling rapid genome sequencing and genetic information analysis.
- AlphaFold achieves over 90% accuracy in protein structure prediction, significantly reducing the need for lengthy experimental procedures.
- AI technologies can process petabytes of omics data, helping scientists decode complex biological systems and extensive biological datasets.
- AI has improved diagnostic accuracy rates to above 95%, such as identifying specific cancer types from pathology slides, enhancing reliability in diagnostics.
- In personalized medicine, AI synthesizes genomics, proteomics, and patient histories to develop tailored treatments, potentially increasing patient survival rates by 10-30%.
- AI accelerates biomarker discovery 50 times faster than conventional methods, expediting the transition from research to clinical applications.
- Incorporating AI in bioinformatics can reduce research expenses by up to 40%, lowering financial barriers to groundbreaking discoveries.
- AI-guided CRISPR technology achieves up to 90% success rates, minimizing unintended effects and improving the safety of gene-editing processes.
- Neural networks attain 84% accuracy in predicting protein amino acid positioning, nearing the theoretical accuracy limit of 88-90%.
- Linear support vector machines have demonstrated a 95.8% success rate in classifying cancer types using RNA sequencing data, showcasing high precision.
- Training advanced AI models like AlphaFold2 requires significant computational power, equivalent to 100-200 GPUs running continuously for several weeks.
- The volume of genomic data doubles approximately every 2.5 years, emphasizing the need for powerful AI tools to manage the expanding information.
- AI-driven drug repurposing studies have identified promising drug interactions, with some molecules showing potential efficacy against proteins linked to Alzheimer’s disease.
- A Big Data and AI-powered platform analyzed a database of over 3,790,000 startups and scaleups worldwide, broadening research capabilities.
- A global Startup Heat Map mapped 4,351 biotech startups and scaleups, illustrating their widespread global distribution.
- US-based startup Arpeggio Bio uses an AI-driven RNA platform to decode complex RNA time series data effectively.
- Swedish startup DeepTrait applies AI in genomics to analyze genomic data precisely and identify gene markers with high accuracy.
Emerging Trends in AI for Bioinformatics
- AI Integration in Biomanufacturing: Biomanufacturing has been transformed through AI, enabling the production of medical products, therapies, and materials using biological systems. AI optimizes bioprocessing steps, making production more cost-effective, scalable, and sustainable. Innovations in cell culture technologies, fermentation processes, and recombinant production are enhanced with AI-powered data analytics and automation, aligning perfectly with Industry 4.0 paradigms.
- Advancements in Synthetic Biology: AI is accelerating progress in synthetic biology by improving standardization and reproducibility of genetic manipulation methods. AI contributes to computational drug design, optimization of metabolic pathways, and designing novel enzymes or genetic circuits. This has expanded applications across industries such as pharmaceuticals, materials science, and food processing.
- Enhanced Bioinformatics Data Analysis: Bioinformatics remains central to modern biological research. AI enables efficient data extraction from biological information through algorithms and advanced software. Tasks such as data storage, retrieval, manipulation, and modeling are enhanced by AI, advancing personalized medicine, genomic exploration, and microbiome research.
- Software and Tools Development: AI-driven advancements are improving bioinformatics software tools, enhancing usability, accessibility, and analytical power. Applications include protein structure prediction, microbiome role analysis, and developing predictive models for environmental and health studies. AI supports the creation of efficient computational frameworks capable of handling the massive volumes of biological data generated daily.
Key Use Cases of AI in Bioinformatics
- Bioinformatics Data Analysis: AI enables advanced analysis of DNA sequencing and gene expression data. Machine learning algorithms, such as neural networks, extract patterns and predict outcomes from vast datasets. These insights help researchers decode biological systems and diseases more effectively.
- Image Analysis in Biology: AI-driven machine learning models are increasingly used for biological image analysis, overcoming human bias and errors. These tools improve precision in cell classification, tissue segmentation, and understanding disease mechanisms at the cellular level, advancing areas like pathology and molecular biology.
- AI in Drug Repurposing: AI is transforming drug repurposing by analyzing large datasets of drug properties and biological interactions. This allows researchers to identify existing drugs that can be repurposed for new therapeutic applications, significantly accelerating discovery and reducing costs.
- AI for Cancer Research: AI integrates multi-omics data (e.g., gene expression, DNA methylation, molecular information) to uncover cancer subtypes and potential therapeutic targets. These advanced methodologies provide new insights into cancer biology and enhance personalized treatment strategies.
- DNA Storage and Computing: AI is assisting researchers in leveraging DNA for data storage and computation. By harnessing DNA’s unique properties, vast amounts of information can be encoded, stored, and retrieved with high efficiency. AI algorithms optimize the encoding process, ensuring accuracy and efficiency of data management.
Challenges in AI for Bioinformatics
- Data Complexity and Volume: The rapid increase in biological data poses significant challenges due to its high complexity, dimensionality, and heterogeneity. Managing and analyzing such vast datasets requires advanced AI models. However, these models often demand substantial computational resources, which can limit their deployment and efficiency, particularly in resource-constrained environments.
- Explainability and Transparency: As AI becomes more integral to bioinformatics, the need for explainable models grows. Many AI applications rely on deep learning models, often described as “black-box” systems due to their lack of transparency. This opacity can undermine trust and accountability, especially in sensitive fields like healthcare and scientific research. Developing interpretable AI systems is crucial to ensure users understand and trust the decision-making processes.
- Ethical and Bias Considerations: Ethical challenges arise when AI models are trained on non-representative datasets, potentially perpetuating existing biases. This can lead to skewed scientific results or unfair healthcare outcomes. Ensuring diverse and inclusive data is essential for fostering fairness, accuracy, and ethical integrity in AI-driven bioinformatics solutions.
- Integration with Traditional Methods: Combining AI with traditional bioinformatics methods involves both technical and cultural challenges. On the technical side, integrating AI requires an overhaul of existing frameworks, which can be resource-intensive. Culturally, fostering collaboration among interdisciplinary teams with different research approaches is essential. Overcoming these challenges will enable AI to unlock its full potential in advancing bioinformatics research and applications.
Opportunities in AI for Bioinformatics
- Enhanced Discovery and Innovation: AI is revolutionizing bioinformatics by automating complex research processes and enabling rapid analysis of massive datasets. This capability is transformative in areas like drug discovery and genetic research, where AI outpaces traditional methods in speed and efficiency. By accelerating research timelines, AI opens new avenues for groundbreaking discoveries that were previously unattainable.
- Precision Medicine: AI is advancing precision medicine by tailoring treatments to patients’ unique genetic profiles. By analyzing intricate biological data, AI identifies the most effective treatment protocols, enhancing treatment efficacy and minimizing adverse reactions. This personalized approach represents a major leap forward in healthcare outcomes and patient care.
- Resource Efficiency: AI optimizes resource utilization in bioinformatics by automating routine tasks and data analyses. This automation allows researchers to focus on strategic priorities, improving productivity and reducing the overall time and cost of experimental procedures. AI-driven resource efficiency ensures that scientific efforts are both effective and streamlined.
- Collaboration and Open Science: AI promotes collaboration and supports the concept of open science. Digital platforms and shared data repositories facilitate seamless information sharing across scientific disciplines and geographies. This interconnected environment fosters global cooperation, broadens research perspectives, and accelerates scientific advancements by making data more accessible and actionable for a diverse scientific community.
Recent Developments in AI for Bioinformatics
- February 2024: QIAGEN (Germany) acquired Cytoneuron, a Singapore-based company specializing in AI-driven single-cell RNA sequencing data analysis. This acquisition enhances QIAGEN’s bioinformatics capabilities, strengthening efforts in advanced drug discovery.
- January 2024: India’s Strand Life Sciences announced the beta release of Medius OS, an AI-powered drug discovery platform. Designed to streamline target identification, molecule generation, and virtual screening, it aims to accelerate drug development timelines and bring new treatments to market faster.
- December 2023: Insilico Medicine (USA) launched its AI platform, PandaOmics, which leverages deep learning to analyze multi-omics data. This innovative tool enables the discovery of new drug targets and biomarkers with improved efficiency.
- October 2023: SOPHiA GENETICS (Switzerland) partnered with Microsoft to develop AI-enhanced solutions for genomic data analysis. Hosted on Microsoft’s cloud platform, the collaboration enhances scalability and accessibility for clinical genomic applications.
- August 2023: SOPHiA GENETICS introduced the SOPHiA AI Clinical Exome, an AI-driven platform that enhances clinical exome analysis and reporting. By analyzing genetic data, it identifies disease-causing variants, enabling faster and more accurate diagnoses for medical professionals.
- July 2023: Thermo Fisher Scientific completed the acquisition of Biomatics, a leader in cloud-based life sciences data management. This move bolsters Thermo Fisher’s AI-driven bioinformatics capabilities, providing advanced tools for biological data management and analytics.
- June 2023: QIAGEN partnered with NVIDIA to develop AI-powered solutions for next-generation sequencing (NGS) data analysis. By integrating NVIDIA’s Clara AI platform and computing hardware, this collaboration accelerates data processing, supporting breakthroughs in precision medicine and drug discovery.
Key Players Analysis
- Fios Genomics: Fios Genomics provides advanced data analysis solutions for drug discovery and development across pharmaceuticals and academia. Their expertise in analyzing multi-variant datasets (NGS, proteomics, metabolomics) delivers biologically relevant insights. A strategic partnership with Charles River Laboratories further strengthens their ability to provide integrated solutions that accelerate research outcomes.
- Source BioScience: Source BioScience integrates AI into genomic and precision medicine. The acquisition of LDPath Ltd for £18.5 million underscores their focus on expanding AI-driven pathology services. These initiatives streamline diagnostic processes, reduce backlogs, and enhance healthcare delivery in both private and NHS sectors.
- Eurofins Scientific: Eurofins Scientific excels in next-generation sequencing (NGS) and AI-driven bioinformatics solutions. Their ISO-certified and GLP-accredited services include sample preparation, library generation, and complex data analysis, supporting genomic projects across various scientific disciplines in Europe.
- Thermo Fisher Scientific: Thermo Fisher leverages AI in bioinformatics through platforms like the Ion Torrent Genexus System, which automates NGS workflows from sample preparation to data analysis. This integration improves efficiency, reduces manual intervention, and accelerates genomic research and healthcare innovations.
- Insilico Medicine: Insilico Medicine is a leader in AI-driven drug discovery, utilizing its Pharma.AI platform for end-to-end drug development. The company advanced INS018_055 into Phase II clinical trials, targeting idiopathic pulmonary fibrosis, showcasing AI’s ability to reduce drug development costs and timelines.
- Paige AI Inc.: Paige AI, in collaboration with Microsoft, leverages Microsoft Azure for AI-powered digital pathology solutions. Their work enhances cancer diagnosis accuracy by enabling precise and rapid analysis of pathology data, positioning Paige as a global leader in clinical AI applications.
- SomaLogic Operating Co. Inc.: SomaLogic specializes in AI-driven proteomics through its SomaScan platform, which measures thousands of proteins in a single assay. Enhanced by AI, this platform integrates multi-omic data for biomarker discovery. A merger with Standard BioTools further expands their technological capabilities.
- SOPHiA GENETICS: SOPHiA GENETICS revolutionizes genomic data analysis through its SOPHiA DDM™ platform, powered by AI. The platform enhances genomic testing accuracy and efficiency, particularly for identifying hereditary cancers. With operations in over 70 countries and analysis of 1.5 million genomic profiles, SOPHiA GENETICS drives precision medicine globally.
- QIAGEN: QIAGEN utilizes AI to enhance NGS capabilities through its QCI Interpret software, supporting clinical exome analysis. Additionally, QIAGEN’s AI-derived biomedical knowledge base, comprising 640 million biomedical relationships, accelerates drug discovery by identifying causal links between genes, diseases, and treatments.
- Strand Life Sciences: Strand Life Sciences focuses on AI-driven genomic research and precision medicine. Their AI-powered platform, StrandNGS, enhances data analysis and integration, providing actionable insights for healthcare. The company aims to democratize precision medicine by making advanced genomic tools accessible worldwide.
Conclusion
The AI in bioinformatics market is experiencing robust growth, driven by technological advancements, decreasing sequencing costs, and increased funding from public and private sectors. Key players, such as Thermo Fisher Scientific, Illumina, and Qiagen, are driving innovation through strategic collaborations.
AI technologies, particularly in machine learning, are revolutionizing genomic data analysis, enhancing disease understanding, and accelerating drug discovery. Despite challenges related to data complexity and ethical concerns, ongoing innovations and investments are expected to address these obstacles, further fueling market expansion. The continued integration of AI will play a crucial role in advancing personalized medicine and bioinformatics research globally.
Discuss your needs with our analyst
Please share your requirements with more details so our analyst can check if they can solve your problem(s)
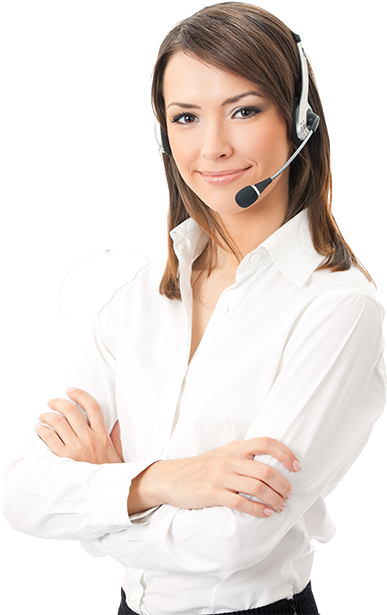