Table of Contents
Introduction
Global Healthcare Fraud Analytics Market size is expected to be worth around USD 20.4 Billion by 2033 from USD 2.5 Billion in 2023, growing at a CAGR of 23.5% during the forecast period from 2024 to 2033.
Healthcare fraud detection involves several key practices, including auditing of accounts, medical claims, and healthcare funds. The detection of fraudulent activities, such as misuse of healthcare funds and insurance fraud, is crucial in the healthcare sector. These fraudulent activities often include falsifying data by healthcare professionals, filing multiple claims for the same patient through different providers, and billing for unprovided services.
Fraud analytics plays a vital role in identifying and preventing these illicit activities. It utilizes data analysis techniques, including data mining and predictive analytics, to discover patterns indicating potential fraud. This approach not only helps in detecting fraudulent transactions and identity theft but also supports organizations in taking immediate action to prevent losses.
The healthcare fraud analytics market is growing, offering solutions that enable healthcare organizations to identify and mitigate fraudulent claims and other deceptive activities efficiently. This innovative and cost-effective method is crucial for reducing healthcare waste and abuse, ensuring that resources are used appropriately and ethically in the industry.
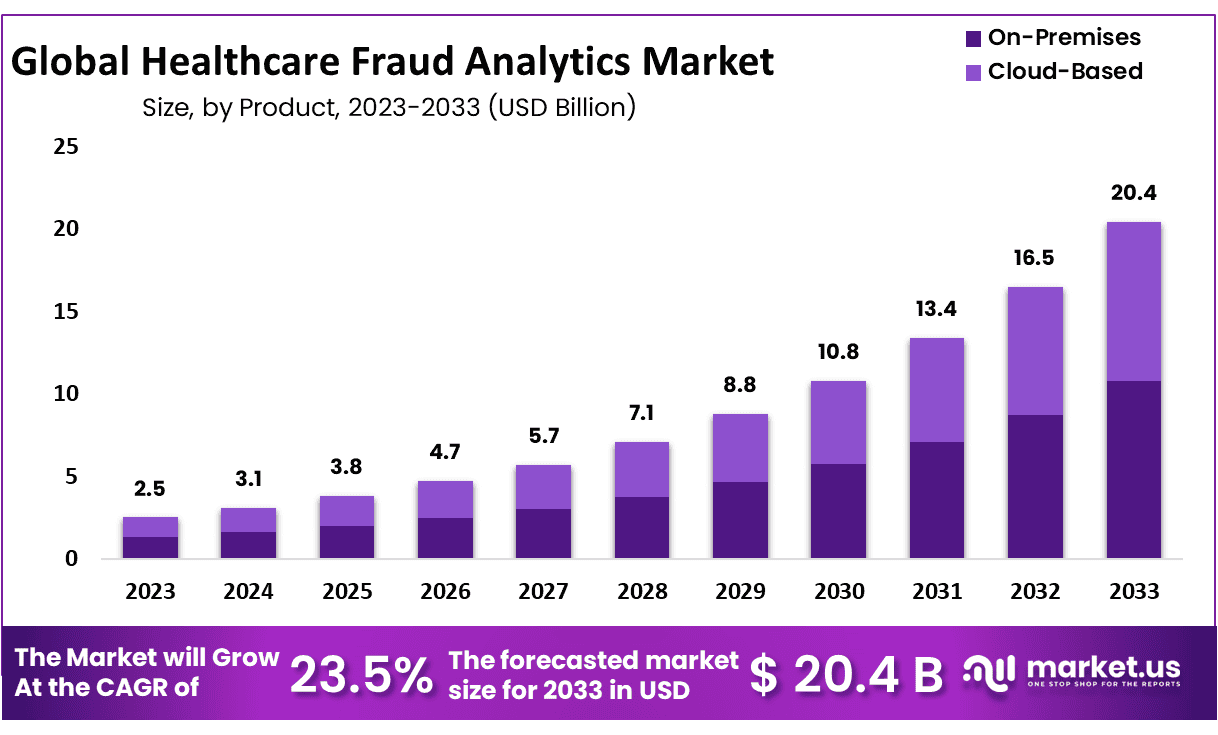
Key Takeaways
- Market Size: Global Healthcare Fraud Analytics Market size is expected to be worth around USD 20.4 Billion by 2033 from USD 2.5 Billion in 2023.
- Market Growth: The market growing at a CAGR of 23.5% during the forecast period from 2024 to 2033.
- Type Analysis: Base on type analysis, Descriptive analytics held approximately 41.2% market share in 2023.
- Delivery Model Analysis: In 2023, on-premise segment held the 52.9% of revenue share.
- Application Analysis: In 2023, the insurance claims review segment dominate over 36.5%.
- End-Use Analysis: Public and government agencies dominated the healthcare fraud analytics market in 2023 with over 40.8%.
- Regional Analysis: North America held approximately 37.8% of the market and holding USD 0.945 Billion revenue in 2023.
- Scope and Importance: The market focuses on detecting and preventing fraudulent activities in healthcare, including claim abuses and insurance fraud, which can significantly drain resources.
- Technological Advancements: Innovations in predictive analytics, data mining, and machine learning are enhancing the ability to identify and prevent fraud more efficiently and effectively.
- Economic Benefits: By reducing instances of fraud, these analytics solutions help conserve valuable healthcare resources, ultimately reducing costs for providers and patients.
Healthcare Fraud Analytics Statistics
- In Fiscal Year 2018, the Department of Justice recovered approximately $2.3 billion from healthcare fraud judgments and settlements.
- During the same period, 1,139 criminal investigations into healthcare fraud were conducted by the Department of Justice.
- Healthcare fraud typically accounts for an estimated 3% to 10% of the total annual healthcare expenditures in the United States.
- The FBI successfully disrupted 812 operational criminal fraud organizations and dismantled the criminal hierarchy of more than 207 healthcare fraud criminal enterprises in 2018.
- Over 2 million Americans have been victims of medical identity theft, which often leads to falsified health records and significant financial issues.
- A psychiatrist involved in a fraud scheme falsely claimed over $20 million in disability payments by entering false diagnoses between 2002 and January 2016.
- In one notable case, the profit from a “pill mill” pain management clinic exceeded $30,000 per day, with the physician overseeing the operation seeing up to 60 patients daily and writing more than 18,000 prescriptions for hydrocodone over approximately two years.
- Individuals associated with this clinic were sentenced to 35 years in prison for their roles in the operation.
- Steven Wasserman, involved in a case under the False Claims Act, paid $26.1 million to resolve allegations without admitting liability for billing Medicare for medically unnecessary services.
- An Ohio cardiologist was sentenced to 20 years in federal prison for causing unnecessary coronary procedures, overbilling Medicare and other insurers by $29 million.
- In a significant legal outcome, a psychiatrist from Miami-Dade was ordered to pay $50 million in restitution for his involvement in a fraudulent scheme involving false psychiatric diagnoses.
Healthcare Fraud Analytics Type Analysis
- Descriptive Analytics: In the realm of Healthcare Fraud Analytics, Descriptive Analytics serves as the foundational layer. It primarily focuses on summarizing historical data to determine what has happened in the past. By aggregating and mining past data, healthcare organizations can identify patterns of fraudulent activities or inconsistencies. This form of analytics is crucial for generating comprehensive reports that depict trends and anomalies in healthcare billing and administration, thereby aiding in the quick identification of potential fraud cases.
- Prescriptive Analytics: Prescriptive Analytics in healthcare fraud goes a step beyond predictive capabilities by not only forecasting outcomes but also suggesting actions to prevent future fraud. This advanced form of analytics uses algorithms and machine learning techniques to provide specific recommendations aimed at stopping fraud before it occurs. By analyzing the patterns and outcomes of past incidents, prescriptive analytics can guide proactive measures, tailoring strategies to address vulnerabilities within healthcare systems effectively.
- Predictive Analytics: Predictive Analytics is pivotal in preempting fraudulent activities in healthcare. It utilizes statistical models and forecast techniques to identify the likelihood of future fraud based on historical data. This analytical approach helps in detecting anomalies and suspicious patterns that deviate from normal behavior, potentially indicating fraudulent activities. The predictive insights gained enable healthcare providers and insurers to implement safeguards and audit checks before significant losses occur, thereby enhancing the integrity of healthcare operations and reducing financial risks associated with fraud.
Emerging Trends
- Shift from Reactive to Proactive Approaches: The focus has shifted from a “pay-and-chase” model to proactive fraud prevention using data analytics and predictive modeling to assess claims before payment.
- Utilization of Big Data: There is increased use of big data to develop insights in biomedical research and provide personalized medicine, enhancing security around sensitive data like medical records and insurance claims.
- Predictive Analytics: Predictive analytics are increasingly employed to create machine learning models that predict disease likelihood and provide real-time alerts to medical staff for better patient care management.
- Artificial Intelligence (AI) and Machine Learning (ML): AI and ML are used to detect unusual patterns that might indicate fraud, optimizing the monitoring and analysis of healthcare claims.
- Enhanced Data Privacy Measures: With rising cybersecurity threats, there’s a strong emphasis on protecting patient data, employing more stringent security measures to prevent breaches that lead to fraud.
- Advanced Training and Education: Training programs are being developed to better equip healthcare providers and analysts with the skills necessary to detect and prevent fraud at its source.
- Integration of Interdisciplinary Skills: There’s a growing demand for professionals with a blend of healthcare knowledge and analytical skills to effectively tackle fraud.
- Expanding Legal Frameworks and Regulations: Regulatory bodies are strengthening laws and penalties related to healthcare fraud to deter malicious activities and ensure stricter compliance.
- Community-based Fraud Detection Initiatives: Initiatives that encourage community and whistleblower involvement in detecting fraud are being enhanced, offering incentives for reporting fraudulent activities.
- Global Collaboration for Fraud Prevention: International cooperation among healthcare providers, payers, and governments is increasing to combat fraud on a global scale.
Use Cases
- Detecting False Claims: Analytical tools can identify patterns indicating claims for services that were never rendered or upcoded claims, where services provided are billed at a higher rate than what was actually performed.
- Preventing Medical Identity Theft: Systems can analyze unusual patterns that may indicate identity theft, helping to prevent wrongful charges and protect patient information.
- Identifying Unnecessary Procedures: Analytics can help flag claims for unnecessary procedures, which not only cost money but also potentially put patients at risk.
- Improving Billing Accuracy: By analyzing billing data, providers can identify errors in real-time and correct them before claims are submitted, reducing the likelihood of fraudulent claims.
- Monitoring Provider Practices: Data analytics can monitor healthcare providers’ billing patterns to identify outliers or unusual practices that may suggest fraudulent activities.
- Enhancing Regulatory Compliance: Healthcare organizations can use data analytics to ensure compliance with healthcare regulations and standards, helping to avoid legal issues and penalties.
- Streamlining Audits: Automated analytics can streamline the audit process by quickly sifting through massive data sets to identify discrepancies or anomalous billing patterns.
- Optimizing Healthcare Operations: By identifying inefficiencies and fraudulent practices, healthcare analytics can help optimize operations, leading to cost savings and improved patient care.
- Enhancing Patient Safety: Analytics can detect potentially harmful practices, such as the prescription of unnecessary medications or procedures, thereby enhancing patient safety.
- Supporting Clinical Decisions: Advanced analytics can support clinical decision-making by providing comprehensive data insights, which can lead to better patient outcomes.
Conclusion
The healthcare fraud analytics market is crucial in combating fraud within the healthcare sector, offering significant economic and operational benefits by identifying and preventing fraudulent activities. Leveraging technologies such as data mining, predictive analytics, AI, and machine learning, these solutions enhance the detection and prevention of fraud, thereby conserving valuable healthcare resources. With a robust growth projection and a shift towards proactive fraud prevention methods, the industry is set to expand significantly, driven by technological advancements and increasing global collaboration. This strategic approach not only reduces costs but also improves patient safety and healthcare delivery efficiency.
Discuss your needs with our analyst
Please share your requirements with more details so our analyst can check if they can solve your problem(s)
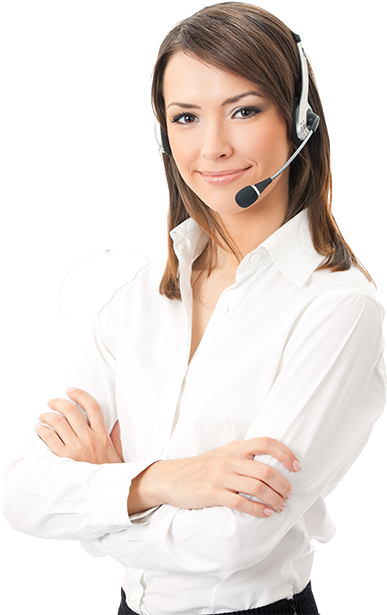