Table of Contents
Introduction
The Global Generative AI in Clinical Trials Market is anticipated to grow from USD 140.5 billion in 2022 to USD 1,135.3 billion by 2032, demonstrating a notable compound annual growth rate of 23.8%. This growth is largely fueled by the integration of generative AI into clinical trials, significantly enhancing research and development. By expediting data generation and processing, this technology shortens the timeline for drug trials, enabling quicker market introduction of new pharmaceuticals. The rapid synthesis and analysis of vast data sets improve the accuracy of trial outcomes and streamline the knowledge transfer process from research to manufacturing.
Generative AI revolutionizes patient engagement and monitoring in clinical trials by automating routine tasks such as data collection and patient interactions. This automation reduces error risk and allows healthcare providers to concentrate on critical decision-making and patient care. Moreover, generative AI creates sophisticated models that simulate human biological processes, aiding the exploratory stages of drug development and reducing real-world trial risks and costs.
The broader adoption of generative AI is driven by its potential to significantly cut development timelines and costs while enhancing the safety and accuracy of clinical research. This technology is becoming indispensable in transforming clinical trials, promising more rapid and precise development of medical treatments. As companies continue to harness these advanced capabilities, the landscape of clinical research is set to evolve dramatically.
Recent strategic developments highlight the industry’s momentum. In April 2024, Microsoft announced a partnership with Cognizant, investing $1 billion to expand generative AI use in enterprise operations, including healthcare. This collaboration leverages Microsoft’s Copilot in Microsoft 365 and GitHub to drive AI innovation. In March 2024, Google launched MedLM, a new healthcare-focused generative AI series for enhancing AI’s capacity to handle diverse healthcare data, thus improving health assessments.
In October 2023, Tencent Holdings Ltd. supported Baichuan, a Chinese AI startup, in a significant funding round that raised $300 million, bringing Baichuan’s valuation to over $1 billion. This investment, also backed by Alibaba Group and Xiaomi Corp., positions Baichuan to compete with major industry players like Microsoft and OpenAI, indicating a robust competitive landscape in the generative AI sector. This surge in investment underscores the growing recognition of generative AI’s transformative impact on various industries, including healthcare.
Key Takeaways
- The Generative AI in Clinical Trials Market is anticipated to rise from USD 140.5 billion in 2022 to USD 1135.3 billion by 2032.
- A notable compound annual growth rate (CAGR) of 23.8% is expected during the forecast period.
- Generative AI is transforming clinical trials by streamlining patient recruitment, trial design, data analysis, and drug development.
- This technology significantly boosts clinical trial efficiency and helps in cost reduction.
- The growth of this market is largely due to the efficiency and productivity gains from generative AI algorithms.
- However, challenges like regulatory uncertainties and issues with data access and privacy hinder its broad adoption.
- Generative AI shows great potential in improving clinical trial design and enhancing the accuracy of outcome predictions.
- Variational Autoencoders, holding a 49% market share, along with Generative Adversarial Networks, are crucial in creating realistic patient data.
- North America leads this market with robust healthcare infrastructure and supportive regulation.
- The Asia Pacific region is expected to witness significant growth, driven by increasing healthcare investments.
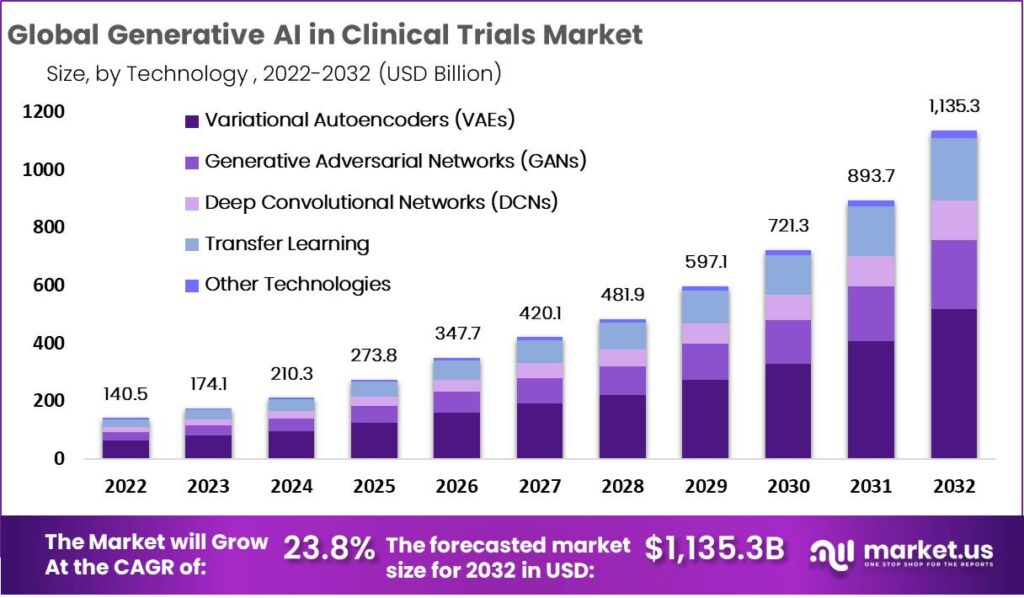
Generative AI in Clinical Trials Statistics
- Generative AI can cut drug discovery costs by up to 70%, enhancing affordability in pharmaceutical development.
- Traditional clinical trial protocols take up to 28 weeks to finalize; AI can significantly speed this process.
- AI in protocol authoring can save up to $50 million per protocol by streamlining the design phase.
- It costs between $100,000 and $150,000 to retain a participant in clinical trials; AI seeks to optimize participant engagement and reduce dropout rates.
- The application of AI enhances the efficiency of processing adverse events by 40-45%, improving safety monitoring.
- AI reduces the time needed to identify and recruit patients by 40%, making the trial start-up process faster.
- The average cost of recruiting a patient for clinical trials reaches around $100,000, a major expenditure that AI aims to lower.
- Clinical trials typically last 6 to 7 years; AI-driven methods promise to shorten this timeline.
- Total patient recruitment expenses can be as high as $1.2 billion, underscoring the need for more efficient strategies like AI.
- Using AI, adverse event reports have surged by 400%, indicating improved reporting and monitoring systems.
- AI can decrease the number of control patients needed by 20% to 50%, potentially reducing trial sizes and costs.
- One-third of a clinical trial’s duration is spent on patient recruitment, highlighting a key area for AI integration.
- Approximately 20% of trials fail due to inadequate participant numbers, an issue AI recruitment tools aim to address.
- AI capabilities allow for cleaning data from over 30,000 patients in under two days, showcasing its speed and scale.
- AI-driven predictive models can foresee trial milestones and lower patient dropout rates, ensuring more reliable study outcomes.
- In one study, AI summarized and reviewed data across 95 clinical trials, demonstrating its capability to handle large datasets.
- Generative AI facilitates accelerated drug discovery by analyzing extensive datasets to formulate new hypotheses.
- By simulating various trial scenarios, AI optimizes clinical trial designs, taking into account diverse patient characteristics and treatment plans.
- AI enables the creation of ‘synthetic’ control groups from existing data, enhancing the precision of trial outcomes.
- Personalized treatment plans generated by AI analyze genetic data to identify optimal patient subgroups for targeted therapies.
- Adaptive trials powered by AI can modify protocols in real-time based on new data, increasing the likelihood of successful outcomes.
- Developing a new drug usually costs about $2.3 billion and takes up to 12 years; AI has the potential to reduce both time and financial investments.
- Nearly 90% of clinical trials face failure due to inefficiencies, a statistic AI is poised to improve.
- Generative AI increases the initial assessment accuracy of drug targets by up to 30%, refining early-stage drug development.
- AI-driven marketing strategies can lower costs by 30-50%, streamlining the promotional activities for new drugs.
- AI can reduce the time for preparing clinical study reports by half, originally taking over eight weeks.
- Regulatory submission processes can be expedited by 40% through AI, enhancing the speed to market for new treatments.
- Initial implementations of AI solutions can show results in just 1-2 months, with further refinements taking an additional two months.
Emerging Trends
- Integration Across Drug Development Phases: Generative AI is revolutionizing the entire drug development spectrum—from discovery through to clinical trials and patient safety monitoring. This technology plays a crucial role in enhancing biological and molecular insights, significantly accelerating the preclinical discovery phase. It achieves this by automating complex data analysis and simulation processes, which traditionally take considerable time and resources, thus speeding up the transition from lab to clinical trials.
- Optimization of Clinical Trial Design: Generative AI is reshaping clinical trial structures by improving the efficiency of data management and protocol formulation. This technology aids in pinpointing the most suitable patient groups and refining eligibility criteria. Consequently, trials become more targeted and streamlined, enhancing the overall efficiency and accuracy of medical research, and potentially reducing the time and cost associated with bringing new therapies to market.
- Enhanced Patient Engagement: Through the development of sophisticated tools, generative AI is poised to transform patient interaction within clinical trials. These tools offer tailored information and support, enhancing communication between researchers and participants. Improved engagement and support not only help in retaining participants throughout the trial but also enhance the quality of data collected, leading to more reliable study outcomes.
- Advances in Predictive and Conversational Analytics: Generative AI is advancing analytics in clinical trials by providing deeper, more actionable insights. This includes predictive analytics that forecast trial outcomes and conversational AI that facilitates real-time decision-making. These technologies enable more dynamic interactions within clinical settings, aiding medical professionals in making informed decisions and strategizing effectively based on ongoing patient data.
- Digital and Virtual Integration in Research: The integration of digital twins and virtual reality powered by generative AI is setting new standards in clinical research methodologies. These technologies allow for more immersive and extensive research environments, which can simulate patient responses more accurately and offer detailed insights into drug effects and safety profiles. This digital approach not only enriches the research experience but also aims to enhance the precision and expansiveness of clinical studies.
Use Cases
- Automated Protocol Generation: Generative AI significantly enhances efficiency in clinical trials by automating the creation of vital documents such as study protocols and clinical reports. This technology streamlines the trial setup and execution process, allowing for quicker start times and more structured management of trial procedures. By reducing manual input and errors, generative AI ensures that all necessary documentation is accurately produced and compliant with regulatory standards, thereby expediting the overall drug development process.
- Patient Data Simulations: Generative AI employs advanced modeling to simulate digital representations of patients, predicting health progressions throughout clinical trials. This capability allows researchers to anticipate various outcomes and understand potential trial results without needing direct patient interventions. Such simulations provide valuable insights into drug efficacy and safety, improving the decision-making process in developing treatment protocols and enhancing patient safety during trials.
- Real-time Data Analysis: Utilizing generative AI for real-time data analysis transforms clinical trial management. This technology analyzes extensive datasets from wearable technology, lab results, and real-world data to predict crucial trial milestones and patient responses. Enhanced predictive capabilities lead to more effective management of trials and better alignment of trial objectives with patient outcomes, ultimately increasing the likelihood of successful drug development.
- Enhancing Diversity in Trials: Generative AI plays a pivotal role in promoting diversity within clinical trials. By identifying and recruiting a broad spectrum of patient demographics, AI ensures the representation of diverse populations. This inclusivity is crucial as it addresses historical disparities in clinical research and enhances the relevance and applicability of trial results across different demographic groups, thereby improving the reliability and acceptance of medical innovations.
- Regulatory Compliance and Documentation: Generative AI is increasingly instrumental in managing complex regulatory documentation and compliance requirements in clinical trials. This technology automates the handling of intricate regulatory procedures, significantly reducing the administrative burden on research teams. Faster processing and adherence to compliance standards not only speed up the approval process but also enhance the integrity and reliability of the trial results, facilitating smoother transitions from trial phases to market launch.
Conclusion
In conclusion, the integration of generative AI into clinical trials marks a transformative shift in the pharmaceutical landscape. This technology streamlines research by automating data analysis and protocol generation, significantly shortening the drug development timeline. As generative AI continues to enhance patient engagement and improve the accuracy of trial outcomes, its adoption is set to increase efficiency and reduce costs across the industry. Despite facing challenges like data privacy and regulatory compliance, the potential of generative AI to revolutionize clinical trials remains substantial. With continued investment and technological advances, generative AI is poised to redefine how medical treatments are developed, promising faster and more effective healthcare solutions.
Discuss your needs with our analyst
Please share your requirements with more details so our analyst can check if they can solve your problem(s)
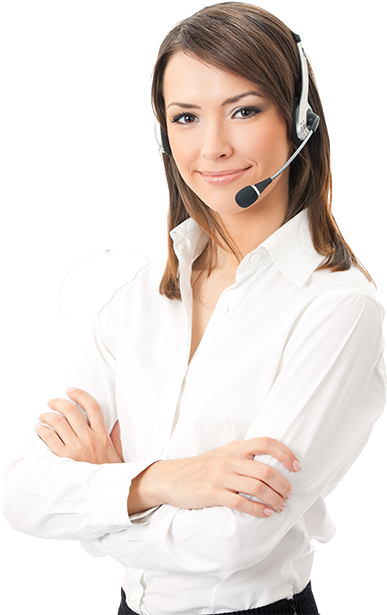