Table of Contents
Introduction
Global AI in Cancer Diagnosis Market is growing rapidly. It is expected to reach USD 1943.6 million by 2033, up from USD 175.3 million in 2023. This represents a compound annual growth rate (CAGR) of 27.2% from 2024 to 2033. With a market share over 58.4%, North America held a strong lead in 2023, reaching USD 102.3 Million in revenue.
This expansion highlights the pivotal role of artificial intelligence (AI) in transforming cancer diagnostics, driven by the growing incidence of cancer and the urgent need for precise and efficient diagnostic methods.
Key drivers of this market growth include the escalating global cancer rates, which demand advanced solutions for early detection and accurate diagnosis. North America is anticipated to maintain its market dominance, due to its high cancer incidence and the substantial adoption of AI technologies in healthcare diagnostics. AI integration significantly boosts the accuracy, speed, and efficiency of cancer diagnosis, enabling timely treatment decisions and personalized care.
Recent developments in the sector emphasize its dynamic nature and potential for innovation. Strategic partnerships between leading healthcare providers and technology companies are enhancing AI-driven diagnostic tools, which help clinicians deliver more effective care. These collaborations are crucial for developing and refining AI applications that aim to improve diagnostic precision, minimize delays, and ultimately enhance patient outcomes.
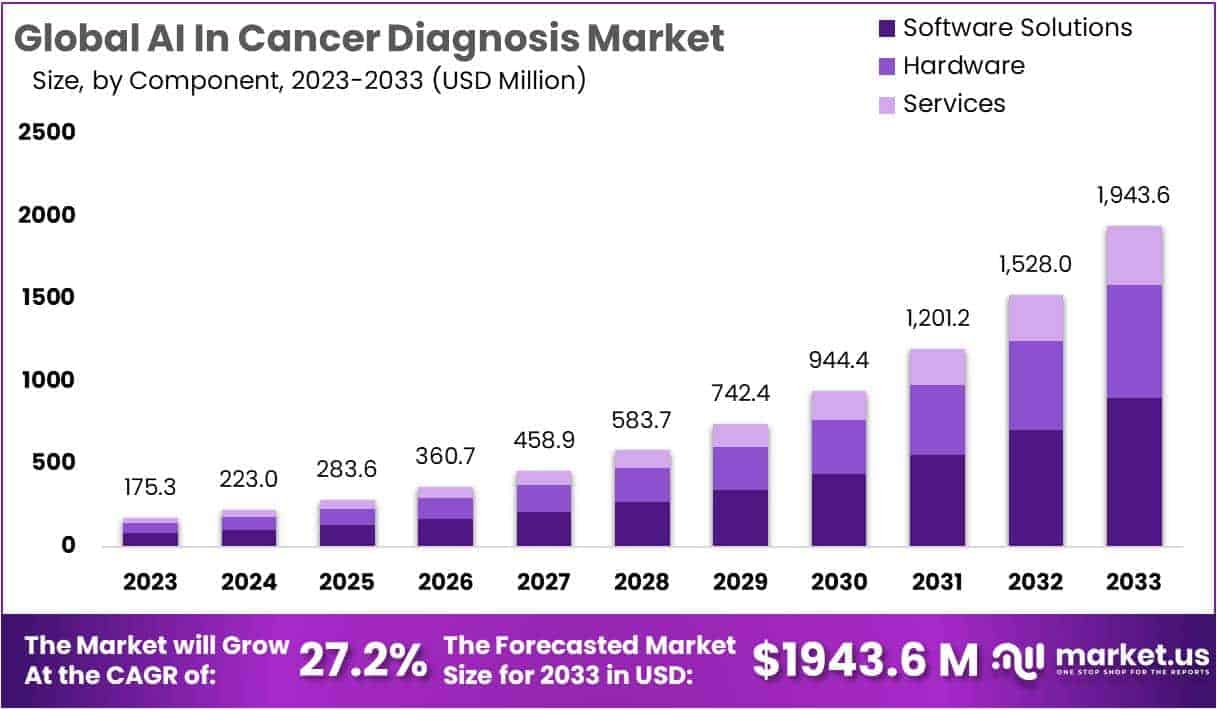
However, the market faces challenges such as the need for extensive data sets to train AI algorithms, high integration costs, and complex regulatory environments. Despite these obstacles, ongoing advancements in AI technology and an increased focus on personalized medicine are expected to promote the widespread adoption of AI in cancer diagnosis. This signifies a promising new phase of innovation and improved care within the oncology sector.
The AI in cancer diagnosis market stands at a critical point, with significant growth opportunities and the potential to profoundly impact healthcare delivery. Marked by technological progress, strategic alliances, and a dedicated focus on enhancing diagnostic processes, the sector is poised to contribute to better patient outcomes and more efficient healthcare systems.
Key Takeaways
- The AI in Cancer Diagnostics market generated a revenue of USD 175.3 million with a CAGR of 27.2%. It is expected to reach USD 1943.6 million by 2033.
- In 2023, software solutions were the highest contributor based on components, holding a market share of 46.5%.
- Among cancer types, the ‘other cancers’ segment generated the most revenue.
- Hospitals remained the primary end-users of AI for cancer diagnosis, securing a market share of 61.0%.
- Region-wise, North America maintained its stronghold on the market, contributing USD 102.3 million in revenue.
AI In Cancer Diagnosis Statistics
- Cancer Incidence and Mortality Rates
- GLOBOCAN 2020 data reported a global annual incidence of 19.3 million new cancer cases and 10 million deaths, with lung cancer being the primary cause of cancer-related deaths at 1.8 million annually.
- In 2023, the U.S. is projected to see 1.9 million new cancer cases and 609,820 deaths, averaging 5370 new cases and 1670 deaths daily.
- Globally, every minute, 37 individuals are diagnosed with cancer, and over 19 succumb to the disease, according to Global Cancer Observatory statistics.
- AI Investments and Innovations in Cancer Diagnosis
- The U.S. is set to invest over $2 billion in AI research by 2025 for infectious disease medicine, significantly up from $463 million in 2019.
- A notable breakthrough from the University of Pittsburgh in July 2020 saw the development of an AI system that diagnoses prostate cancer with 98% accuracy, displaying high specificity and sensitivity.
- Efficiency and Accuracy of AI Models
- Various AI models like the random forest model and auto-encoders have shown remarkable accuracy rates of 96% across different cancer types.
- Artificial neural networks have been successfully used for predicting pancreatic cancer with an 85% AUC score and for distinguishing lung cancer types with a 97% accuracy.
- Techniques such as DNA methylation have improved cancer classification significantly, reclassifying 70% of cases with up to 93% accuracy in identifying 82 brain tumor types.
- AI in Digital Pathology and Radiology
- In the UK, digital pathology is gaining traction with 60% of institutions having access to digital scanners as of 2018.
- AI models have shown potential in reducing pathologists’ workloads by up to 57.2%, as demonstrated by studies utilizing large datasets for lung cancer detection and mammogram analysis.
- AI-enhanced triage systems in mammography have enabled effective rule-out thresholds, significantly reducing the need for radiologist reviews.
- Advancements in AI-Driven Cancer Detection
- Recent AI software has achieved a 100% detection rate for melanoma and a 99.5% accuracy rate for identifying all skin cancers.
- The combination of AI with digital mammography and Digital Breast Tomosynthesis (DBT) has improved cancer detection rates and reduced recall rates, showing the promising integration of AI in routine cancer screening.
- Challenges and Future Directions
- Despite the advancements, the integration of AI in cancer diagnosis faces challenges like the need for large data sets, high costs, and regulatory complexities.
- The emphasis remains on improving early detection rates, as evidenced by the NHS’s goal to increase early cancer diagnosis to 75% by 2028, highlighting the critical role of AI in achieving this target.
Emerging Trends
- Enhanced Precision in Brain Tumor Diagnosis: The application of Artificial Intelligence (AI) in brain tumor diagnosis is transforming medical imaging by integrating AI with cutting-edge imaging technologies. This synergy enables clinicians to more accurately and swiftly identify tumor boundaries during surgical procedures. AI’s real-time analysis helps distinguish cancerous tissues from healthy ones, thereby not only improving surgical outcomes but also refining treatment planning. This advancement is crucial for enhancing the precision of interventions and customizing patient care.
- Predictive Capabilities in Medical Imaging: AI is extending beyond the traditional scope of radiological analysis by offering predictive insights directly from medical imaging data, such as MRIs. This includes the capacity to predict patient outcomes, identify tumor characteristics, and even detect specific gene mutations from imaging scans without the need for invasive biopsies. This shift toward non-invasive diagnostic methods represents a significant stride in personalizing treatment plans and advancing patient-centric care, marking a revolutionary step in oncology.
- AI’s Role in Cancer Research and Drug Development: Significant collaborations, such as those between the National Cancer Institute (NCI) and various research entities, highlight the role of AI in furthering cancer research and drug development. AI technologies are being employed to conduct detailed genomic profiling of tumors and to accelerate the pace of drug discovery. This includes AI-driven analysis of cancer proteins, which aids in the development of targeted therapies. Additionally, AI’s ability to predict treatment responses and monitor patient progress is improving the efficacy of ongoing treatments and surveillance strategies.
Use Cases
- Enhancing Early Detection in Pancreatic Cancer: Artificial Intelligence (AI) is redefining early detection in pancreatic cancer by utilizing expansive patient data to assess risk factors and predict disease onset. This proactive approach identifies individuals at high risk, enabling timely and targeted interventions that could markedly improve outcomes. AI’s deep analytical capabilities provide a vital tool in navigating the complexities of pancreatic cancer, promoting early diagnosis and tailored treatment plans.
- Revolutionizing Precision Oncology: AI is spearheading a revolution in precision oncology, where treatments are increasingly tailored to the genetic details of individual tumors. By analyzing genetic and proteomic information, AI facilitates the creation of personalized treatment protocols that directly target the unique aspects of a patient’s cancer. This precision approach not only enhances the effectiveness of treatments but also minimizes unnecessary side effects, heralding a new era in cancer care.
- Advancing Diagnostic Accuracy Through Medical Imaging: AI significantly improves the accuracy and speed of cancer diagnoses through advanced medical imaging. By integrating AI with imaging technologies, clinicians can detect cancerous tissues and anomalies with enhanced precision. AI’s ability to quickly interpret complex imaging data ensures faster diagnosis, crucial for effective cancer management. This technology becomes an invaluable asset in the early detection and accurate diagnosis of various cancers, improving the chances of successful treatment.
- Fostering Collaborative Synergy Between AI and Healthcare Professionals: The application of AI in cancer diagnosis promotes a synergistic relationship between technology and medical professionals. AI supplements the clinical expertise of healthcare providers with comprehensive data analysis and pattern recognition capabilities. This partnership enhances diagnostic processes and decision-making, ensuring high-quality patient care. By combining the strengths of both AI and human judgment, the diagnostic accuracy and efficiency in cancer care are significantly enhanced.
AI In Cancer Diagnosis Key Player Analysis
- EarlySign: EarlySign has formed a strategic partnership with Roche to utilize its LungFlag technology, a machine learning tool that sifts through extensive patient datasets to predict early lung cancer occurrence. This collaboration is pivotal in enhancing early detection and personalized treatment strategies, potentially boosting survival rates for one of the most lethal cancers globally. EarlySign’s initiative highlights the trend of using AI to improve diagnostic accuracy, promote early disease detection, and customize patient management, ultimately optimizing outcomes and reducing healthcare costs.
- Cancer Center.ai: Cancer Center.ai is propelling advancements in AI for cancer diagnosis with its platform that integrates medical imaging with AI to refine the diagnostic process. The latest update, CancerCenter.ai 2.0, introduced an enhanced interface and new functionalities designed to support pathologists and radiologists by streamlining image analysis and management. This platform’s user-friendly tools and faster operation are vital for professionals in high-stakes medical environments.
- Microsoft and Paige: Microsoft’s collaboration with Paige focuses on developing the world’s largest image-based AI models for digital pathology and oncology. Leveraging Microsoft’s AI capabilities and Azure cloud infrastructure, this partnership aims to bolster Paige’s digital pathology solutions, enhancing the accuracy and efficiency of cancer diagnostics. This global initiative promises significant improvements in patient care by facilitating faster and more precise cancer diagnoses in labs and hospitals worldwide.
- Flatiron Health: Flatiron Health is leading AI integration in cancer diagnosis, particularly through expanding its real-world data (RWD) capabilities. Its partnerships with the FDA and the National Comprehensive Cancer Network (NCCN) focus on utilizing AI-driven analytics to advance real-world evidence (RWE) applications, optimize clinical trial designs, and improve outcomes for diverse cancer populations. These collaborations are crucial for refining RWE for regulatory use and closing significant evidence gaps in oncology.
- PathAI: PathAI is enhancing cancer diagnosis through its advanced AI-driven pathology technology. The expansion of its PathExplore tool to include additional oncology indicators aids in the comprehensive analysis of tumor environments, crucial for the development of targeted cancer therapies. PathAI’s collaboration with Quest Diagnostics, involving the acquisition of PathAI Diagnostics’ assets, seeks to accelerate the adoption of digital and AI pathology across the U.S., thus enhancing the quality and efficiency of cancer diagnostics.
- Therapixel: Therapixel, specializing in AI for medical imaging, significantly advances breast cancer detection with its MammoScreen technology. This tool, applicable to both 2D and 3D mammography, aids radiologists by enhancing diagnostic accuracy and reducing reading times. With FDA endorsement and a recent partnership with Qure.ai, Therapixel’s technology is crucial for enhancing early detection practices, improving patient outcomes through early and accurate diagnosis.
- Tempus: Tempus leverages its AI-driven precision medicine platform to make notable advancements in cancer diagnosis. By utilizing a comprehensive library of multimodal data, Tempus’s analytics identify potential therapeutic options and clinical trial matches, greatly influencing personalized cancer care. Its collaboration with major pharmaceutical companies like Pfizer integrates AI into drug development, furthering innovations in oncology therapeutics.
- Paige AI: Paige AI’s partnership with Microsoft centers on creating the largest image-based AI models to revolutionize digital pathology. Their efforts aim to make advanced AI diagnostics more accessible, supporting Paige’s FDA-approved AI tools that simplify complex diagnostic processes and enhance the accuracy and efficiency of cancer diagnostics globally.
- Kheiron Medical Technologies Limited: Kheiron Medical is a standout in the AI in cancer diagnosis sector, especially in breast cancer screening, with its Mia platform. This technology improves the accuracy and efficiency of breast cancer screenings and is demonstrated to enhance detection rates significantly. With regulatory approvals across multiple regions and plans to expand functionalities, Kheiron is set to further support breast screening clinics.
- SkinVision: SkinVision leverages AI for early skin cancer detection using an algorithm trained on a vast database of skin spot images. Achieving a 95% accuracy rate, SkinVision provides quick and reliable skin cancer risk assessments, continuously enhancing its diagnostic capabilities through ongoing clinical validation and research partnerships, underscoring its commitment to improving early detection and treatment outcomes.
Conclusion
The AI in cancer diagnostics sector is rapidly evolving, characterized by significant technological advancements and strategic collaborations that enhance diagnostic accuracy and patient outcomes. With North America leading in market revenue and adoption rates, the integration of AI in healthcare is revolutionizing oncology by improving early detection and enabling personalized treatment strategies.
Despite challenges such as the need for extensive datasets and high costs, the potential for AI to transform cancer care is immense. Continued innovation and investment in this field are likely to drive further adoption and development, promising better diagnostics and more effective cancer treatments in the future.
Discuss your needs with our analyst
Please share your requirements with more details so our analyst can check if they can solve your problem(s)
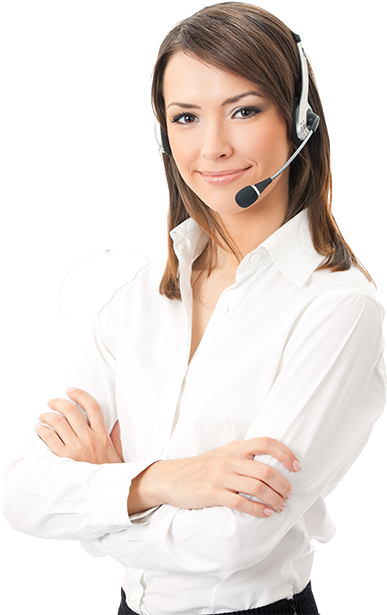